Open Access
ARTICLE
A New Multi-Agent Feature Wrapper Machine Learning Approach for Heart Disease Diagnosis
1 Department of Computer Science, College of Computer Information Technology, American University in the Emirates, 503000, United Arab Emirates
2 College of Computer Science and Information Technology, University of Anbar, Ramadi, 31001, Iraq
3 Faculty of Computer Science and Information Technology, Universiti Tun Hussein Onn Malaysia, Johor, 86400, Malaysia
4 College of Agriculture, Al-Muthanna University, Samawah, 66001, Iraq
5 Department of Software Engineering, College of Computer and Information Sciences, King Saud University, Riyadh, 11451, Saudi Arabia
6 eVIDA Lab, University of Deusto, Bilbao, 48007, Spain
7 Department of Pure Science, Ministry of Education, General Directorate of Curricula, Baghdad, Iraq
8 Department of Medical Laboratory Science, Faculty of Applied Medical Science, King Abdulaziz University, Jeddah, 21589, Saudi Arabia
* Corresponding Author: Mazin Abed Mohammed. Email:
(This article belongs to the Special Issue: Deep Learning Trends in Intelligent Systems)
Computers, Materials & Continua 2021, 67(1), 51-71. https://doi.org/10.32604/cmc.2021.012632
Received 07 July 2020; Accepted 20 October 2020; Issue published 12 January 2021
Abstract
Heart disease (HD) is a serious widespread life-threatening disease. The heart of patients with HD fails to pump sufficient amounts of blood to the entire body. Diagnosing the occurrence of HD early and efficiently may prevent the manifestation of the debilitating effects of this disease and aid in its effective treatment. Classical methods for diagnosing HD are sometimes unreliable and insufficient in analyzing the related symptoms. As an alternative, noninvasive medical procedures based on machine learning (ML) methods provide reliable HD diagnosis and efficient prediction of HD conditions. However, the existing models of automated ML-based HD diagnostic methods cannot satisfy clinical evaluation criteria because of their inability to recognize anomalies in extracted symptoms represented as classification features from patients with HD. In this study, we propose an automated heart disease diagnosis (AHDD) system that integrates a binary convolutional neural network (CNN) with a new multi-agent feature wrapper (MAFW) model. The MAFW model consists of four software agents that operate a genetic algorithm (GA), a support vector machine (SVM), and Naïve Bayes (NB). The agents instruct the GA to perform a global search on HD features and adjust the weights of SVM and BN during initial classification. A final tuning to CNN is then performed to ensure that the best set of features are included in HD identification. The CNN consists of five layers that categorize patients as healthy or with HD according to the analysis of optimized HD features. We evaluate the classification performance of the proposed AHDD system via 12 common ML techniques and conventional CNN models by using a cross-validation technique and by assessing six evaluation criteria. The AHDD system achieves the highest accuracy of 90.1%, whereas the other ML and conventional CNN models attain only 72.3%–83.8% accuracy on average. Therefore, the AHDD system proposed herein has the highest capability to identify patients with HD. This system can be used by medical practitioners to diagnose HD efficiently.Keywords
Cite This Article
Citations
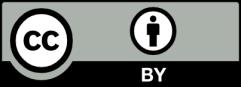