Open Access
ARTICLE
Brainwave Classification for Character-Writing Application Using EMD-Based GMM and KELM Approaches
1 Department of Telecommunication Engineering, Rajamangala University of Technology Isan, Nakhon Ratchasima, 30000, Thailand
2 School of Telecommunication Engineering, Suranaree University of Technology, Nakhon Ratchasima, 30000, Thailand
3 Tianjin Key Laboratory of Cognitive Computing and Application, College of Intelligence and Computing, Tianjin University, Tianjin, 300350, China
4 Department of Materials Science, Chulalongkorn University, Bangkok, 10330, Thailand
* Corresponding Author: Peerapong Uthansakul. Email:
Computers, Materials & Continua 2021, 66(3), 3029-3044. https://doi.org/10.32604/cmc.2021.014433
Received 20 September 2020; Accepted 18 October 2020; Issue published 28 December 2020
Abstract
A brainwave classification, which does not involve any limb movement and stimulus for character-writing applications, benefits impaired people, in terms of practical communication, because it allows users to command a device/computer directly via electroencephalogram signals. In this paper, we propose a new framework based on Empirical Mode Decomposition (EMD) features along with the Gaussian Mixture Model (GMM) and Kernel Extreme Learning Machine (KELM)-based classifiers. For this purpose, firstly, we introduce EMD to decompose EEG signals into Intrinsic Mode Functions (IMFs), which actually are used as the input features of the brainwave classification for the character-writing application. We hypothesize that EMD along with the appropriate IMF is quite powerful for the brainwave classification, in terms of character applications, because of the wavelet-like decomposition without any down sampling process. Secondly, by getting motivated with shallow learning classifiers, we can provide promising performance for the classification of binary classes, GMM and KELM, which are applied for the learning of features along with the brainwave classification. Lastly, we propose a new method by combining GMM and KELM to fuse the merits of different classifiers. Moreover, the proposed methods are validated by using the volunteer-independent 5-fold cross-validation and accuracy as a standard measurement. The experimental results showed that EMD with the proper IMF achieved better results than the conventional discrete wavelet transform (DWT) feature. Moreover, we found that the EMD feature along with the GMM/KELM-based classifier provides the average accuracy of 77.40% and 80.10%, respectively, which could perform better than the conventional methods where we use DWT along with the artificial neural network classifier in order to get the average accuracy of 80.60%. Furthermore, we obtained the improved performance by combining GMM and KELM, i.e., average accuracy of 80.60%. These outcomes exhibit the usefulness of the EMD feature combining with GMM and KELM based classifiers for the brainwave classification in terms of the Character-Writing application, which do not require any limb movement and stimulus.Keywords
Cite This Article
Citations
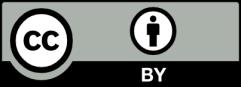