Open Access
ARTICLE
An Abstractive Summarization Technique with Variable Length Keywords as per Document Diversity
1 Department of Software Engineering, Government College University, Faisalabad, Faisalabad, 38000, Pakistan
2 Department of IT and Computer Science, Pak-Austria Fachhochschule Institute of Applied Sciences and Technology, Haripur, Pakistan
3 Department of Computer Science, Islamia College Peshawar, Peshawar, Pakistan
4 Institute of Computing, Kohat University of Science and Technology, Kohat, Pakistan
5 Faculty of Applied Studies, King Abdulaziz University, Jeddah, Saudi Arabia
* Corresponding Author: Muhammad Arif Shah. Email:
Computers, Materials & Continua 2021, 66(3), 2409-2423. https://doi.org/10.32604/cmc.2021.014330
Received 14 September 2020; Accepted 10 October 2020; Issue published 28 December 2020
Abstract
Text Summarization is an essential area in text mining, which has procedures for text extraction. In natural language processing, text summarization maps the documents to a representative set of descriptive words. Therefore, the objective of text extraction is to attain reduced expressive contents from the text documents. Text summarization has two main areas such as abstractive, and extractive summarization. Extractive text summarization has further two approaches, in which the first approach applies the sentence score algorithm, and the second approach follows the word embedding principles. All such text extractions have limitations in providing the basic theme of the underlying documents. In this paper, we have employed text summarization by TF-IDF with PageRank keywords, sentence score algorithm, and Word2Vec word embedding. The study compared these forms of the text summarizations with the actual text, by calculating cosine similarities. Furthermore, TF-IDF based PageRank keywords are extracted from the other two extractive summarizations. An intersection over these three types of TD-IDF keywords to generate the more representative set of keywords for each text document is performed. This technique generates variable-length keywords as per document diversity instead of selecting fixed-length keywords for each document. This form of abstractive summarization improves metadata similarity to the original text compared to all other forms of summarized text. It also solves the issue of deciding the number of representative keywords for a specific text document. To evaluate the technique, the study used a sample of more than eighteen hundred text documents. The abstractive summarization follows the principles of deep learning to create uniform similarity of extracted words with actual text and all other forms of text summarization. The proposed technique provides a stable measure of similarity as compared to existing forms of text summarization.Keywords
Cite This Article
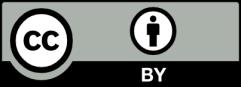
This work is licensed under a Creative Commons Attribution 4.0 International License , which permits unrestricted use, distribution, and reproduction in any medium, provided the original work is properly cited.