Open Access
ARTICLE
A New Class of L-Moments Based Calibration Variance Estimators
1 Department of Mathematics and Statistics, International Islamic University, Islamabad, 46000, Pakistan
2 Department of Mathematics and Statistics, PMAS-Arid Agriculture University, Rawalpindi, 46300, Pakistan
3 Department of Mathematics, King Khalid University, Abha, 62529, Saudi Arabia
4 Statistical Research and Studies Support Unit, King Khalid University, Abha, 62529, Saudi Arabia
5 Department of Mathematics, College of Science, Mustansiriyah University, Baghdad, 10011, Iraq
* Corresponding Author: Usman Shahzad. Email:
Computers, Materials & Continua 2021, 66(3), 3013-3028. https://doi.org/10.32604/cmc.2021.014101
Received 31 August 2020; Accepted 19 October 2020; Issue published 28 December 2020
Abstract
Variance is one of the most important measures of descriptive statistics and commonly used for statistical analysis. The traditional second-order central moment based variance estimation is a widely utilized methodology. However, traditional variance estimator is highly affected in the presence of extreme values. So this paper initially, proposes two classes of calibration estimators based on an adaptation of the estimators recently proposed by Koyuncu and then presents a new class of L-Moments based calibration variance estimators utilizing L-Moments characteristics (L-location, L-scale, L-CV) and auxiliary information. It is demonstrated that the proposed L-Moments based calibration variance estimators are more efficient than adapted ones. Artificial data is considered for assessing the performance of the proposed estimators. We also demonstrated an application related to apple fruit for purposes of the article. Using artificial and real data sets, percentage relative efficiency (PRE) of the proposed class of estimators with respect to adapted ones are calculated. The PRE results indicate to the superiority of the proposed class over adapted ones in the presence of extreme values. In this manner, the proposed class of estimators could be applied over an expansive range of survey sampling whenever auxiliary information is available in the presence of extreme values.Keywords
Cite This Article
Citations
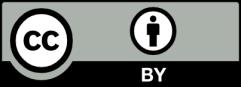
This work is licensed under a Creative Commons Attribution 4.0 International License , which permits unrestricted use, distribution, and reproduction in any medium, provided the original work is properly cited.