Open Access
ARTICLE
An Efficient False-Positive Reduction System for Cerebral Microbleeds Detection
1 Department of Computer Science, COMSATS University Islamabad, Attock Campus, Attock, Pakistan
2 Department of Media Design and Technology, Faculty of Engineering & Informatics, University of Bradford, Bradford, BD7 1AZ, UK
3 Department of Smart Device Engineering, School of Intelligent Mechatronics Engineering, Sejong University, Seoul, South Korea
4 School of Computer Art, College of Art & Technology, Chung-Ang University, Anseong, 17546, South Korea
* Corresponding Author: Muazzam Maqsood. Email:
(This article belongs to the Special Issue: Artificial Intelligence and IoT based intelligent systems using high performance computing for Medical applications.)
Computers, Materials & Continua 2021, 66(3), 2301-2315. https://doi.org/10.32604/cmc.2021.013966
Received 27 August 2020; Accepted 26 September 2020; Issue published 28 December 2020
Abstract
Cerebral Microbleeds (CMBs) are microhemorrhages caused by certain abnormalities of brain vessels. CMBs can be found in people with Traumatic Brain Injury (TBI), Alzheimer’s disease, and in old individuals having a brain injury. Current research reveals that CMBs can be highly dangerous for individuals having dementia and stroke. The CMBs seriously impact individuals’ life which makes it crucial to recognize the CMBs in its initial phase to stop deterioration and to assist individuals to have a normal life. The existing work report good results but often ignores false-positive’s perspective for this research area. In this paper, an efficient approach is presented to detect CMBs from the Susceptibility Weighted Images (SWI). The proposed framework consists of four main phases (i) making clusters of brain Magnetic Resonance Imaging (MRI) using k-mean classifier (ii) reduce false positives for better classification results (iii) discriminative feature extraction specific to CMBs (iv) classification using a five layers convolutional neural network (CNN). The proposed method is evaluated on a public dataset available for 20 subjects. The proposed system shows an accuracy of 98.9% and a 1.1% false-positive rate value. The results show the superiority of the proposed work as compared to existing states of the art methods.Keywords
Cite This Article
Citations
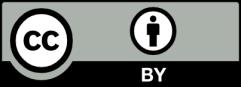
This work is licensed under a Creative Commons Attribution 4.0 International License , which permits unrestricted use, distribution, and reproduction in any medium, provided the original work is properly cited.