Open Access
ARTICLE
Statistical Inference of Chen Distribution Based on Two Progressive Type-II Censoring Schemes
Department of Mathematics & Statistics, Faculty of Science, Taif University, Taif, 21944, Saudi Arabia
* Corresponding Author: Hassan M. Aljohani. Email:
Computers, Materials & Continua 2021, 66(3), 2797-2814. https://doi.org/10.32604/cmc.2021.013489
Received 08 August 2020; Accepted 29 October 2020; Issue published 28 December 2020
Abstract
An inverse problem in practical scientific investigations is the process of computing unknown parameters from a set of observations where the observations are only recorded indirectly, such as monitoring and controlling quality in industrial process control. Linear regression can be thought of as linear inverse problems. In other words, the procedure of unknown estimation parameters can be expressed as an inverse problem. However, maximum likelihood provides an unstable solution, and the problem becomes more complicated if unknown parameters are estimated from different samples. Hence, researchers search for better estimates. We study two joint censoring schemes for lifetime products in industrial process monitoring. In practice, this type of data can be collected in fields such as the medical industry and industrial engineering. In this study, statistical inference for the Chen lifetime products is considered and analyzed to estimate underlying parameters. Maximum likelihood and Bayes’ rule are both studied for model parameters. The asymptotic distribution of maximum likelihood estimators and the empirical distributions obtained with Markov chain Monte Carlo algorithms are utilized to build the interval estimators. Theoretical results using tables and figures are adopted through simulation studies and verified in an analysis of the lifetime data. We briefly describe the performance of developed methods.Keywords
Cite This Article
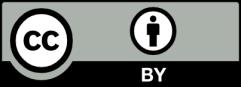
This work is licensed under a Creative Commons Attribution 4.0 International License , which permits unrestricted use, distribution, and reproduction in any medium, provided the original work is properly cited.