Open Access
ARTICLE
Trade-Off between Efficiency and Effectiveness: A Late Fusion Multi-View Clustering Algorithm
1 National University of Defence Technology, Changsha, China
2 Department of Mathematics, Faculty of Science, University of Sargodha, Sargodha, Pakistan
* Corresponding Author: Jianzhuang Lu. Email:
Computers, Materials & Continua 2021, 66(3), 2709-2722. https://doi.org/10.32604/cmc.2021.013389
Received 05 August 2020; Accepted 12 September 2020; Issue published 28 December 2020
Abstract
Late fusion multi-view clustering (LFMVC) algorithms aim to integrate the base partition of each single view into a consensus partition. Base partitions can be obtained by performing kernel k-means clustering on all views. This type of method is not only computationally efficient, but also more accurate than multiple kernel k-means, and is thus widely used in the multi-view clustering context. LFMVC improves computational efficiency to the extent that the computational complexity of each iteration is reduced from O(n3) to O(n) (where n is the number of samples). However, LFMVC also limits the search space of the optimal solution, meaning that the clustering results obtained are not ideal. Accordingly, in order to obtain more information from each base partition and thus improve the clustering performance, we propose a new late fusion multi-view clustering algorithm with a computational complexity of O(n2). Experiments on several commonly used datasets demonstrate that the proposed algorithm can reach quickly convergence. Moreover, compared with other late fusion algorithms with computational complexity of O(n), the actual time consumption of the proposed algorithm does not significantly increase. At the same time, comparisons with several other state-of-the-art algorithms reveal that the proposed algorithm also obtains the best clustering performance.Keywords
Cite This Article
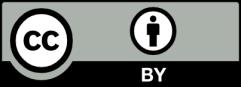
This work is licensed under a Creative Commons Attribution 4.0 International License , which permits unrestricted use, distribution, and reproduction in any medium, provided the original work is properly cited.