Open Access
ARTICLE
Prediction of COVID-19 Cases Using Machine Learning for Effective Public Health Management
1 Department of Computer Sciences, Kinnaird College for Women, Lahore, 54000, Pakistan
2 Department of Information Systems, College of Computer and Information Sciences, Jouf University, Sakaka, Aljouf, 72341, Saudi Arabia
3 Division of Computer Science & Information Technology, University of Education, Lahore, 54000, Pakistan
4 School of Computer Science, National College of Business Administration & Economics, Lahore, 54000, Pakistan
5 Department of Clinical Laboratory Sciences, College of Applied Medical Sciences, Jouf University, Sakaka, Aljouf, 72341, Saudi Arabia
* Corresponding Authors: Fahad Ahmad. Email: ,
(This article belongs to the Special Issue: Mathematical aspects of the Coronavirus Disease 2019 (COVID-19): Analysis and Control)
Computers, Materials & Continua 2021, 66(3), 2265-2282. https://doi.org/10.32604/cmc.2021.013067
Received 24 July 2020; Accepted 30 September 2020; Issue published 28 December 2020
Abstract
COVID-19 is a pandemic that has affected nearly every country in the world. At present, sustainable development in the area of public health is considered vital to securing a promising and prosperous future for humans. However, widespread diseases, such as COVID-19, create numerous challenges to this goal, and some of those challenges are not yet defined. In this study, a Shallow Single-Layer Perceptron Neural Network (SSLPNN) and Gaussian Process Regression (GPR) model were used for the classification and prediction of confirmed COVID-19 cases in five geographically distributed regions of Asia with diverse settings and environmental conditions: namely, China, South Korea, Japan, Saudi Arabia, and Pakistan. Significant environmental and non-environmental features were taken as the input dataset, and confirmed COVID-19 cases were taken as the output dataset. A correlation analysis was done to identify patterns in the cases related to fluctuations in the associated variables. The results of this study established that the population and air quality index of a region had a statistically significant influence on the cases. However, age and the human development index had a negative influence on the cases. The proposed SSLPNN-based classification model performed well when predicting the classes of confirmed cases. During training, the binary classification model was highly accurate, with a Root Mean Square Error (RMSE) of 0.91. Likewise, the results of the regression analysis using the GPR technique with Matern 5/2 were highly accurate (RMSE = 0.95239) when predicting the number of confirmed COVID-19 cases in an area. However, dynamic management has occupied a core place in studies on the sustainable development of public health but dynamic management depends on proactive strategies based on statistically verified approaches, like Artificial Intelligence (AI). In this study, an SSLPNN model has been trained to fit public health associated data into an appropriate class, allowing GPR to predict the number of confirmed COVID-19 cases in an area based on the given values of selected parameters. Therefore, this tool can help authorities in different ecological settings effectively manage COVID-19.Keywords
Cite This Article
Citations
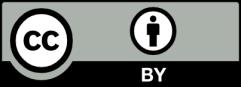
This work is licensed under a Creative Commons Attribution 4.0 International License , which permits unrestricted use, distribution, and reproduction in any medium, provided the original work is properly cited.