Open Access
ARTICLE
Deep Learning in DXA Image Segmentation
1 School of Computational Science, Korea Institute for Advanced Study (KIAS), 85 Hoegiro, Dongdaemun-gu, Seoul, 02455, South Korea
2 Department of Unmanned Vehicle Engineering, Sejong University, 209, Neungdong-ro, Gwangjin-gu, Seoul, 05006, South Korea
3 Department of Software, Gachon University, Seongnam, 13120, South Korea
* Corresponding Author: Jooyoung Lee. Email:
Computers, Materials & Continua 2021, 66(3), 2587-2598. https://doi.org/10.32604/cmc.2021.013031
Received 22 July 2020; Accepted 26 August 2020; Issue published 28 December 2020
Abstract
Many existing techniques to acquire dual-energy X-ray absorptiometry (DXA) images are unable to accurately distinguish between bone and soft tissue. For the most part, this failure stems from bone shape variability, noise and low contrast in DXA images, inconsistent X-ray beam penetration producing shadowing effects, and person-to-person variations. This work explores the feasibility of using state-of-the-art deep learning semantic segmentation models, fully convolutional networks (FCNs), SegNet, and U-Net to distinguish femur bone from soft tissue. We investigated the performance of deep learning algorithms with reference to some of our previously applied conventional image segmentation techniques (i.e., a decision-tree-based method using a pixel label decision tree [PLDT] and another method using Otsu’s thresholding) for femur DXA images, and we measured accuracy based on the average Jaccard index, sensitivity, and specificity. Deep learning models using SegNet, U-Net, and an FCN achieved average segmentation accuracies of 95.8%, 95.1%, and 97.6%, respectively, compared to PLDT (91.4%) and Otsu’s thresholding (72.6%). Thus we conclude that an FCN outperforms other deep learning and conventional techniques when segmenting femur bone from soft tissue in DXA images. Accurate femur segmentation improves bone mineral density computation, which in turn enhances the diagnosing of osteoporosis.Keywords
Cite This Article
Citations
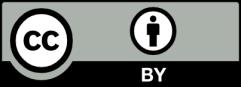
This work is licensed under a Creative Commons Attribution 4.0 International License , which permits unrestricted use, distribution, and reproduction in any medium, provided the original work is properly cited.