Open Access
ARTICLE
Dealing with Imbalanced Dataset Leveraging Boundary Samples Discovered by Support Vector Data Description
1 Graduate School of Information, Production and Systems, Waseda University, Tokyo, Japan
2 Institute of Research and Development, Duy Tan University, Da Nang, 550000, Vietnam
3 Faculty of Information Technology, Duy Tan University, Da Nang, 550000, Vietnam
4 Department of Computer Science, Nourabad Mamasani Branch, Islamic Azad University, Mamasani, Iran
5 School of Mathematics, Thapar Institute of Engineering and Technology, Deemed University, Patiala, Punjab, 147004, India
6 Computer Science Department, College of Computer and Information Sciences, Al Imam Mohammad Ibn Saud Islamic University, Riyadh, Saudi Arabia
7 Computer Science Department, Faculty of Applied Science, Taiz University, Taiz, Yemen
8 Fractional Calculus, Optimization and Algebra Research Group, Faculty of Mathematics and Statistics, Ton Duc Thang University, Ho Chi Minh City, Vietnam
9 Fakulti Teknologi dan Sains Maklumat, Universiti Kebangsaan Malaysia, Selangor, Malaysia
* Corresponding Author: Hamïd Parvïn. Email:
Computers, Materials & Continua 2021, 66(3), 2691-2708. https://doi.org/10.32604/cmc.2021.012547
Received 03 July 2020; Accepted 08 August 2020; Issue published 28 December 2020
Abstract
These days, imbalanced datasets, denoted throughout the paper by ID, (a dataset that contains some (usually two) classes where one contains considerably smaller number of samples than the other(s)) emerge in many real world problems (like health care systems or disease diagnosis systems, anomaly detection, fraud detection, stream based malware detection systems, and so on) and these datasets cause some problems (like under-training of minority class(es) and over-training of majority class(es), bias towards majority class(es), and so on) in classification process and application. Therefore, these datasets take the focus of many researchers in any science and there are several solutions for dealing with this problem. The main aim of this study for dealing with IDs is to resample the borderline samples discovered by Support Vector Data Description (SVDD). There are naturally two kinds of resampling: Under-sampling (U-S) and over-sampling (O-S). The O-S may cause the occurrence of over-fitting (the occurrence of over-fitting is its main drawback). The U-S can cause the occurrence of significant information loss (the occurrence of significant information loss is its main drawback). In this study, to avoid the drawbacks of the sampling techniques, we focus on the samples that may be misclassified. The data points that can be misclassified are considered to be the borderline data points which are on border(s) between the majority class(es) and minority class(es). First by SVDD, we find the borderline examples; then, the data resampling is applied over them. At the next step, the base classifier is trained on the newly created dataset. Finally, we compare the result of our method in terms of Area Under Curve (AUC) and F-measure and G-mean with the other state-of-the-art methods. We show that our method has better results than the other state-of-the-art methods on our experimental study.Keywords
Cite This Article
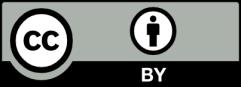
This work is licensed under a Creative Commons Attribution 4.0 International License , which permits unrestricted use, distribution, and reproduction in any medium, provided the original work is properly cited.