Open Access
ARTICLE
An Automated Penetration Semantic Knowledge Mining Algorithm Based on Bayesian Inference
1 State Key Laboratory of Mathematical Engineering and Advanced Computing, Zhengzhou, 450000, China
2 National Engineering Technology Research Center of the Digital Switching System, Zhengzhou, 450000, China
3 NUS Business School, National University of Singapore, Singapore, 119077, Singapore
* Corresponding Author: Yichao Zang. Email:
Computers, Materials & Continua 2021, 66(3), 2573-2585. https://doi.org/10.32604/cmc.2021.012220
Received 20 June 2021; Accepted 19 July 2020; Issue published 28 December 2020
Abstract
Mining penetration testing semantic knowledge hidden in vast amounts of raw penetration testing data is of vital importance for automated penetration testing. Associative rule mining, a data mining technique, has been studied and explored for a long time. However, few studies have focused on knowledge discovery in the penetration testing area. The experimental result reveals that the long-tail distribution of penetration testing data nullifies the effectiveness of associative rule mining algorithms that are based on frequent pattern. To address this problem, a Bayesian inference based penetration semantic knowledge mining algorithm is proposed. First, a directed bipartite graph model, a kind of Bayesian network, is constructed to formalize penetration testing data. Then, we adopt the maximum likelihood estimate method to optimize the model parameters and decompose a large Bayesian network into smaller networks based on conditional independence of variables for improved solution efficiency. Finally, irrelevant variable elimination is adopted to extract penetration semantic knowledge from the conditional probability distribution of the model. The experimental results show that the proposed method can discover penetration semantic knowledge from raw penetration testing data effectively and efficiently.Keywords
Cite This Article
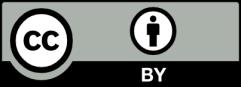
This work is licensed under a Creative Commons Attribution 4.0 International License , which permits unrestricted use, distribution, and reproduction in any medium, provided the original work is properly cited.