Open Access
ARTICLE
Cooperative Channel Assignment for VANETs Based on Dual Reinforcement Learning
1 Beijing Advanced Innovation Center for Big Data and Brain Computing, Beijing, 100191, China
2 School of Transportation Science and Engineering, Beihang University, Beijing, 100191, China
3 Department of Engineering and Design, University of Sussex, Brighton, BN1 9RH, UK
4 Research Institute of Highway Ministry of Transport, Beijing, 100088, China
* Corresponding Author: Kunxian Zheng. Email:
Computers, Materials & Continua 2021, 66(2), 2127-2140. https://doi.org/10.32604/cmc.2020.014484
Received 23 September 2020; Accepted 06 October 2020; Issue published 26 November 2020
Abstract
Dynamic channel assignment (DCA) is significant for extending vehicular ad hoc network (VANET) capacity and mitigating congestion. However, the un-known global state information and the lack of centralized control make channel assignment performances a challenging task in a distributed vehicular direct communication scenario. In our preliminary field test for communication under V2X scenario, we find that the existing DCA technology cannot fully meet the communication performance requirements of VANET. In order to improve the communication performance, we firstly demonstrate the feasibility and potential of reinforcement learning (RL) method in joint channel selection decision and access fallback adaptation design in this paper. Besides, a dual reinforcement learning (DRL)-based cooperative DCA (DRL-CDCA) mechanism is proposed. Specifically, DRL-CDCA jointly optimizes the decision-making behaviors of both the channel selection and back-off adaptation based on a multi-agent dual reinforcement learning framework. Besides, nodes locally share and incorporate their individual rewards after each communication to achieve regional consistency optimization. Simulation results show that the proposed DRL-CDCA can better reduce the one-hop packet delay, improve the packet delivery ratio on average when compared with two other existing mechanisms.Keywords
Cite This Article
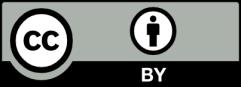