Open Access
ARTICLE
Hajj Crowd Management Using CNN-Based Approach
1 Department of Information Technology, College of Computer, Qassim University, Buraydah, Saudi Arabia
2 Electronic Section, Universiti Kuala Lumpur British Malaysian Institute, Malaysia
3 Department of Electrical Engineering, Onaizah Colleges, Saudi Arabia
4 Department of Electrical and Computer Engineering, International Islamic University, Malaysia
* Corresponding Author: Waleed Albattah. Email:
(This article belongs to the Special Issue: Machine Learning-based Intelligent Systems: Theories, Algorithms, and Applications)
Computers, Materials & Continua 2021, 66(2), 2183-2197. https://doi.org/10.32604/cmc.2020.014227
Received 07 September 2020; Accepted 24 September 2020; Issue published 26 November 2020
Abstract
Hajj as the Muslim holy pilgrimage, attracts millions of humans to Mecca every year. According to statists, the pilgrimage has attracted close to 2.5 million pilgrims in 2019, and at its peak, it has attracted over 3 million pilgrims in 2012. It is considered as the world’s largest human gathering. Safety makes one of the main concerns with regards to managing the large crowds and ensuring that stampedes and other similar overcrowding accidents are avoided. This paper presents a crowd management system using image classification and an alarm system for managing the millions of crowds during Hajj. The image classification system greatly relies on the appropriate dataset used to train the Convolutional neural network (CNN), which is the deep learning technique that has recently attracted the interest of the research community and industry in varying applications of image classification and speech recognition. The core building block of CNN is is a convolutional layer obtained by the getting CNN trained with patches bearing designated features of the trainee mages. The algorithm is implemented, using the Conv2D layers to activate the CNN as a sequential network. Thus, creating a 2D convolution layer having 64 filters and drop out of 0.5 makes the core of a CNN referred to as a set of KERNELS. The aim is to train the CNN model with mapped image data, and to make it available for use in classifying the crowd as heavily-crowded, crowded, semi-crowded, light crowded, and normal. The utility of these results lies in producing appropriate signals for proving helpful in monitoring the pilgrims. Counting pilgrims from the photos will help the authorities to determine the number of people in certain areas. The results demonstrate the utility of agent-based modeling for Hajj pilgrims.Keywords
Cite This Article
Citations
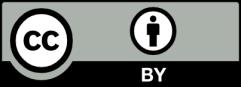
This work is licensed under a Creative Commons Attribution 4.0 International License , which permits unrestricted use, distribution, and reproduction in any medium, provided the original work is properly cited.