Open Access
ARTICLE
Click through Rate Effectiveness Prediction on Mobile Ads Using Extreme Gradient Boosting
College of Computer Science and Information Technology, Imam Abdulrahman bin Faisal University, Dammam, 34221, Saudi Arabia
* Corresponding Author: Nida Aslam. Email:
Computers, Materials & Continua 2021, 66(2), 1681-1696. https://doi.org/10.32604/cmc.2020.013466
Received 18 August 2020; Accepted 12 September 2020; Issue published 26 November 2020
Abstract
Online advertisements have a significant influence over the success or failure of your business. Therefore, it is important to somehow measure the impact of your advertisement before uploading it online, and this is can be done by calculating the Click Through Rate (CTR). Unfortunately, this method is not eco-friendly, since you have to gather the clicks from users then compute the CTR. This is where CTR prediction come in handy. Advertisement CTR prediction relies on the users’ log regarding click information data. Accurate prediction of CTR is a challenging and critical process for e-advertising platforms these days. CTR prediction uses machine learning techniques to determine how much the online advertisement has been clicked by a potential client: The more clicks, the more successful the ad is. In this study we develop a machine learning based click through rate prediction model. The proposed study defines a model that generates accurate results with low computational power consumption. We used four classification techniques, namely K Nearest Neighbor (KNN), Logistic Regression, Random Forest, and Extreme Gradient Boosting (XGBoost). The study was performed on the Click-Through Rate Prediction Competition Dataset. It is a click-through data that is ordered chronologically and was collected over 10 days. Experimental results reveal that XGBoost produced ROC-AUC of 0.76 with reduced number of features.Keywords
Cite This Article
Citations
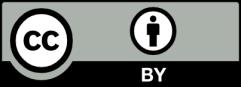
This work is licensed under a Creative Commons Attribution 4.0 International License , which permits unrestricted use, distribution, and reproduction in any medium, provided the original work is properly cited.