Open Access
ARTICLE
Approach for Training Quantum Neural Network to Predict Severity of COVID-19 in Patients
1 Department of Computer Science, Faculty of Computers and Artificial Intelligence, University of Sadat City, Sadat City, 32897, Egypt
2 Faculty of Computers and Artificial Intelligence, Cairo University, 12613, Egypt
3 Faculty of Computers and Information, Zagazig University, 44519, Egypt
4 Faculty of Computers and Information, Kafrelsheikh University, 33516, Egypt
* Corresponding Author: Karam M. Sallam. Email:
Computers, Materials & Continua 2021, 66(2), 1745-1755. https://doi.org/10.32604/cmc.2020.013066
Received 24 July 2020; Accepted 11 September 2020; Issue published 26 November 2020
Abstract
Currently, COVID-19 is spreading all over the world and profoundly impacting people’s lives and economic activities. In this paper, a novel approach called the COVID-19 Quantum Neural Network (CQNN) for predicting the severity of COVID-19 in patients is proposed. It consists of two phases: In the first, the most distinct subset of features in a dataset is identified using a Quick Reduct Feature Selection (QRFS) method to improve its classification performance; and, in the second, machine learning is used to train the quantum neural network to classify the risk. It is found that patients’ serial blood counts (their numbers of lymphocytes from days 1 to 15 after admission to hospital) are associated with relapse rates and evaluations of COVID-19 infections. Accordingly, the severity of COVID-19 is classified in two categories, serious and non-serious. The experimental results indicate that the proposed CQNN’s prediction approach outperforms those of other classification algorithms and its high accuracy confirms its effectiveness.Keywords
Cite This Article
Citations
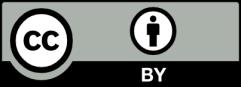
This work is licensed under a Creative Commons Attribution 4.0 International License , which permits unrestricted use, distribution, and reproduction in any medium, provided the original work is properly cited.