Open Access
ARTICLE
A Self-Learning Data-Driven Development of Failure Criteria of Unknown Anisotropic Ductile Materials with Deep Learning Neural Network
1 Department of Aerospace Engineering, Seoul National University, Seoul, 088262, South Korea
2 Institute of Advanced Aerospace Technology, Seoul National University, Seoul, 08826, South Korea
* Corresponding Author: Gun Jin Yun. Email:
Computers, Materials & Continua 2021, 66(2), 1091-1120. https://doi.org/10.32604/cmc.2020.012911
Received 17 July 2020; Accepted 27 August 2020; Issue published 26 November 2020
Abstract
This paper first proposes a new self-learning data-driven methodology that can develop the failure criteria of unknown anisotropic ductile materials from the minimal number of experimental tests. Establishing failure criteria of anisotropic ductile materials requires time-consuming tests and manual data evaluation. The proposed method can overcome such practical challenges. The methodology is formalized by combining four ideas: 1) The deep learning neural network (DLNN)-based material constitutive model, 2) Self-learning inverse finite element (SELIFE) simulation, 3) Algorithmic identification of failure points from the self-learned stress-strain curves and 4) Derivation of the failure criteria through symbolic regression of the genetic programming. Stress update and the algorithmic tangent operator were formulated in terms of DLNN parameters for nonlinear finite element analysis. Then, the SELIFE simulation algorithm gradually makes the DLNN model learn highly complex multi-axial stress and strain relationships, being guided by the experimental boundary measurements. Following the failure point identification, a self-learning data-driven failure criteria are eventually developed with the help of a reliable symbolic regression algorithm. The methodology and the self-learning data-driven failure criteria were verified by comparing with a reference failure criteria and simulating with different materials orientations, respectively.Keywords
Cite This Article
Citations
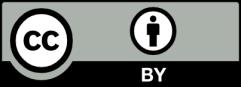