Open Access
ARTICLE
Early Detection of Diabetic Retinopathy Using Machine Intelligence through Deep Transfer and Representational Learning
1 Department of Computer Science and Information Technology, University of Sargodha, Sargodha, 40100, Pakistan and School of Systems and Technology, University of Management and Technology, Lahore, 54782, Pakistan
2 Department of Computer Science, COMSATS University Islamabad, Wah Campus, Islamabad, Pakistan
3 University Hospital Birmingham, Birmingham, UK
4 Shifa International Hospital, Islamabad, Pakistan
5 Department of Computer Science and Engineering, Sejong University, Seoul, Korea
* Corresponding Author: Muhammad Raheel Bhutta. Email:
(This article belongs to the Special Issue: Artificial Intelligence and IoT based intelligent systems using high performance computing for Medical applications.)
Computers, Materials & Continua 2021, 66(2), 1631-1645. https://doi.org/10.32604/cmc.2020.012887
Received 16 July 2020; Accepted 11 September 2020; Issue published 26 November 2020
Abstract
Diabetic retinopathy (DR) is a retinal disease that causes irreversible blindness. DR occurs due to the high blood sugar level of the patient, and it is clumsy to be detected at an early stage as no early symptoms appear at the initial level. To prevent blindness, early detection and regular treatment are needed. Automated detection based on machine intelligence may assist the ophthalmologist in examining the patients’ condition more accurately and efficiently. The purpose of this study is to produce an automated screening system for recognition and grading of diabetic retinopathy using machine learning through deep transfer and representational learning. The artificial intelligence technique used is transfer learning on the deep neural network, Inception-v4. Two configuration variants of transfer learning are applied on Inception-v4: Fine-tune mode and fixed feature extractor mode. Both configuration modes have achieved decent accuracy values, but the fine-tuning method outperforms the fixed feature extractor configuration mode. Fine-tune configuration mode has gained 96.6% accuracy in early detection of DR and 97.7% accuracy in grading the disease and has outperformed the state of the art methods in the relevant literature.Keywords
Cite This Article
Citations
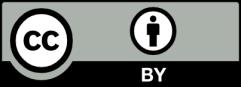