Open Access
ARTICLE
Marker-Based and Marker-Less Motion Capturing Video Data: Person and Activity Identification Comparison Based on Machine Learning Approaches
1 School of Computer Science, National College for Business Administration and Economics, Lahore, 54000, Pakistan
2 Department of Computer Science, Lahore Garrison University, Lahore, 54792, Pakistan
3 Computer Science Department, Umm Al-Qura University, Makkah City, Saudi Arabia
* Corresponding Author: Muhammad Adnan Khan. Email:
Computers, Materials & Continua 2021, 66(2), 1269-1282. https://doi.org/10.32604/cmc.2020.012778
Received 12 July 2020; Accepted 28 August 2020; Issue published 26 November 2020
Abstract
Biomechanics is the study of physiological properties of data and the measurement of human behavior. In normal conditions, behavioural properties in stable form are created using various inputs of subconscious/conscious human activities such as speech style, body movements in walking patterns, writing style and voice tunes. One cannot perform any change in these inputs that make results reliable and increase the accuracy. The aim of our study is to perform a comparative analysis between the marker-based motion capturing system (MBMCS) and the marker-less motion capturing system (MLMCS) using the lower body joint angles of human gait patterns. In both the MLMCS and MBMCS, we collected trajectories of all the participants and performed joint angle computation to identify a person and recognize an activity (walk and running). Using five state of the art machine learning algorithms, we obtained 44.6% and 64.3% accuracy in person identification using MBMCS and MLMCS respectively with an ensemble algorithm (two angles as features). In the second set of experiments, we used six machine learning algorithms to obtain 65.9% accuracy with the k-nearest neighbor (KNN) algorithm (two angles as features) and 74.6% accuracy with an ensemble algorithm. Also, by increasing features (6 angles), we obtained higher accuracy of 99.3% in MBMCS for person recognition and 98.1% accuracy in MBMCS for activity recognition using the KNN algorithm. MBMCS is computationally expensive and if we re-design the model of OpenPose with more body joint points and employ more features, MLMCS (low-cost system) can be an effective approach for video data analysis in a person identification and activity recognition process.Keywords
Cite This Article
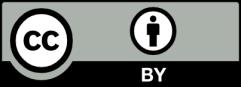
This work is licensed under a Creative Commons Attribution 4.0 International License , which permits unrestricted use, distribution, and reproduction in any medium, provided the original work is properly cited.