Open Access
ARTICLE
A Stacking-Based Deep Neural Network Approach for Effective Network Anomaly Detection
1 Department of Computer and Information Security, Sejong University, Seoul, 05006, Korea
2 Data Science Group, Institute for Basic Science (IBS), Daejeon, 34126, Korea
3 Department of Industrial Engineering, Ulsan National Institute of Science and Technology, Ulsan, 44919, Korea
* Corresponding Author: Sunghoon Lim. Email:
Computers, Materials & Continua 2021, 66(2), 2217-2227. https://doi.org/10.32604/cmc.2020.012432
Received 30 June 2020; Accepted 26 August 2020; Issue published 26 November 2020
Abstract
An anomaly-based intrusion detection system (A-IDS) provides a critical aspect in a modern computing infrastructure since new types of attacks can be discovered. It prevalently utilizes several machine learning algorithms (ML) for detecting and classifying network traffic. To date, lots of algorithms have been proposed to improve the detection performance of A-IDS, either using individual or ensemble learners. In particular, ensemble learners have shown remarkable performance over individual learners in many applications, including in cybersecurity domain. However, most existing works still suffer from unsatisfactory results due to improper ensemble design. The aim of this study is to emphasize the effectiveness of stacking ensemble-based model for A-IDS, where deep learning (e.g., deep neural network [DNN]) is used as base learner model. The effectiveness of the proposed model and base DNN model are benchmarked empirically in terms of several performance metrics, i.e., Matthew’s correlation coefficient, accuracy, and false alarm rate. The results indicate that the proposed model is superior to the base DNN model as well as other existing ML algorithms found in the literature.Keywords
Cite This Article
Citations
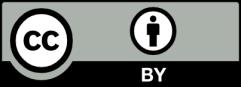
This work is licensed under a Creative Commons Attribution 4.0 International License , which permits unrestricted use, distribution, and reproduction in any medium, provided the original work is properly cited.