Open Access
ARTICLE
A Novel Hybrid Intelligent Prediction Model for Valley Deformation: A Case Study in Xiluodu Reservoir Region, China
1 Research Institute of Geotechnical Engineering, Hohai University, Nanjing, 210098, China
2 Key Laboratory of Ministry of Education for Geomechanics and Embankment Engineering, Hohai University, Nanjing, 210098, China
3 Key Laboratory of Coastal Disaster and Defense, Ministry of Education, Hohai University, Nanjing, 210098, China
4 Department of Civil and Environmental Engineering, University of Waterloo, Waterloo, N2L 3G1, Canada
* Corresponding Author: Weiya Xu. Email:
Computers, Materials & Continua 2021, 66(1), 1057-1074. https://doi.org/10.32604/cmc.2020.012537
Received 03 July 2020; Accepted 25 July 2020; Issue published 30 October 2020
Abstract
The narrowing deformation of reservoir valley during the initial operation period threatens the long-term safety of the dam, and an accurate prediction of valley deformation (VD) remains a challenging part of risk mitigation. In order to enhance the accuracy of VD prediction, a novel hybrid model combining Ensemble empirical mode decomposition based interval threshold denoising (EEMD-ITD), Differential evolutions—Shuffled frog leaping algorithm (DE-SFLA) and Least squares support vector machine (LSSVM) is proposed. The non-stationary VD series is firstly decomposed into several stationary subseries by EEMD; then, ITD is applied for redundant information denoising on special sub-series, and the denoised deformation is divided into the trend and periodic deformation components. Meanwhile, several relevant triggering factors affecting the VD are considered, from which the input features are extracted by Grey relational analysis (GRA). After that, DE-SFLA-LSSVM is separately performed to predict the trend and periodic deformation with the optimal inputs. Ultimately, the two individual forecast components are reconstructed to obtain the final predicted values. Two VD series monitored in Xiluodu reservoir region are utilized to verify the proposed model. The results demonstrate that: (1) Compared with Discrete wavelet transform (DWT), better denoising performance can be achieved by EEMD-ITD; (2) Using GRA to screen the optimal input features can effectively quantify the deformation response relationship to the triggering factors, and reduce the model complexity; (3) The proposed hybrid model in this study displays superior performance on some compared models (e.g., LSSVM, Backward Propagation neural network (BPNN), and DE-SFLA-BPNN) in terms of forecast accuracy.Keywords
Cite This Article
Citations
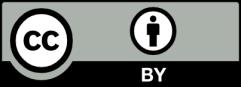
This work is licensed under a Creative Commons Attribution 4.0 International License , which permits unrestricted use, distribution, and reproduction in any medium, provided the original work is properly cited.