Open Access
ARTICLE
A Convolutional Neural Network Classifier VGG-19 Architecture for Lesion Detection and Grading in Diabetic Retinopathy Based on Deep Learning
1 Department of Electronics and Communication Engineering, Pavai College of Technology, Namakkal, 637018, India
2 Department of Electronics and Communication Engineering, Muthayammal Engineering College, Rasipuram, 637408, India
* Corresponding Author: V. Sudha. Email:
Computers, Materials & Continua 2021, 66(1), 827-842. https://doi.org/10.32604/cmc.2020.012008
Received 10 June 2020; Accepted 16 July 2020; Issue published 30 October 2020
Abstract
Diabetic Retinopathy (DR) is a type of disease in eyes as a result of a diabetic condition that ends up damaging the retina, leading to blindness or loss of vision. Morphological and physiological retinal variations involving slowdown of blood flow in the retina, elevation of leukocyte cohesion, basement membrane dystrophy, and decline of pericyte cells, develop. As DR in its initial stage has no symptoms, early detection and automated diagnosis can prevent further visual damage. In this research, using a Deep Neural Network (DNN), segmentation methods are proposed to detect the retinal defects such as exudates, hemorrhages, microaneurysms from digital fundus images and then the conditions are classified accurately to identify the grades as mild, moderate, severe, no PDR, PDR in DR. Initially, saliency detection is applied on color images to detect maximum salient foreground objects from the background. Next, structure tensor is applied powerfully to enhance the local patterns of edge elements and intensity changes that occur on edges of the object. Finally, active contours approximation is performed using gradient descent to segment the lesions from the images. Afterwards, the output images from the proposed segmentation process are subjected to evaluate the ratio between the total contour area and the total true contour arc length to label the classes as mild, moderate, severe, No PDR and PDR. Based on the computed ratio obtained from segmented images, the severity levels were identified. Meanwhile, statistical parameters like the mean and the standard deviation of pixel intensities, mean of hue, saturation and deviation clustering, are estimated through K-means, which are computed as features from the output images of the proposed segmentation process. Using these derived feature sets as input to the classifier, the classification of DR was performed. Finally, a VGG-19 deep neural network was trained and tested using the derived feature sets from the KAGGLE fundus image dataset containing 35,126 images in total. The VGG-19 is trained with features extracted from 20,000 images and tested with features extracted from 5,000 images to achieve a sensitivity of 82% and an accuracy of 96%. The proposed system was able to label and classify DR grades automatically.Keywords
Cite This Article
Citations
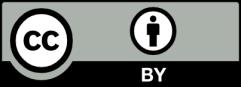