Open Access
ARTICLE
Privacy-Preserving Recommendation Based on Kernel Method in Cloud Computing
1 College of Artificial Intelligence, Nanjing University of Information Science and Technology, Nanjing, 210044, China
2 College of Electronic and Information Engineering, Nanjing University of Information Science and Technology, Nanjing, 210044, China
3 College of Computer and Software, Nanjing University of Information Science and Technology, Nanjing, 210044, China
4 College of Electronic and Information Engineering, Nanjing University of Information Science and Technology, Nanjing, 210044, China
5 International Business Machines Corporation (IBM), NY, USA
* Corresponding Author: Yongjun Ren. Email:
Computers, Materials & Continua 2021, 66(1), 779-791. https://doi.org/10.32604/cmc.2020.010424
Received 04 March 2020; Accepted 08 May 2020; Issue published 30 October 2020
Abstract
The application field of the Internet of Things (IoT) involves all aspects, and its application in the fields of industry, agriculture, environment, transportation, logistics, security and other infrastructure has effectively promoted the intelligent development of these aspects. Although the IoT has gradually grown in recent years, there are still many problems that need to be overcome in terms of technology, management, cost, policy, and security. We need to constantly weigh the benefits of trusting IoT products and the risk of leaking private data. To avoid the leakage and loss of various user data, this paper developed a hybrid algorithm of kernel function and random perturbation method based on the algorithm of non-negative matrix factorization, which realizes personalized recommendation and solves the problem of user privacy data protection in the process of personalized recommendation. Compared to non-negative matrix factorization privacy-preserving algorithm, the new algorithm does not need to know the detailed information of the data, only need to know the connection between each data; and the new algorithm can process the data points with negative characteristics. Experiments show that the new algorithm can produce recommendation results with certain accuracy under the premise of preserving users’ personal privacy.Keywords
Cite This Article
Citations
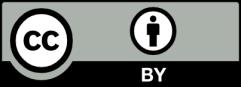
This work is licensed under a Creative Commons Attribution 4.0 International License , which permits unrestricted use, distribution, and reproduction in any medium, provided the original work is properly cited.