Open Access
ARTICLE
Anomaly Classification Using Genetic Algorithm-Based Random Forest Model for Network Attack Detection
Management Information Systems Department, College of Business, King Khalid University, Abha, 61421, Saudi Arabia
* Corresponding Author: Adel Assiri. Email:
Computers, Materials & Continua 2021, 66(1), 767-778. https://doi.org/10.32604/cmc.2020.013813
Received 22 August 2020; Accepted 14 September 2020; Issue published 30 October 2020
Abstract
Anomaly classification based on network traffic features is an important task to monitor and detect network intrusion attacks. Network-based intrusion detection systems (NIDSs) using machine learning (ML) methods are effective tools for protecting network infrastructures and services from unpredictable and unseen attacks. Among several ML methods, random forest (RF) is a robust method that can be used in ML-based network intrusion detection solutions. However, the minimum number of instances for each split and the number of trees in the forest are two key parameters of RF that can affect classification accuracy. Therefore, optimal parameter selection is a real problem in RF-based anomaly classification of intrusion detection systems. In this paper, we propose to use the genetic algorithm (GA) for selecting the appropriate values of these two parameters, optimizing the RF classifier and improving the classification accuracy of normal and abnormal network traffics. To validate the proposed GA-based RF model, a number of experiments is conducted on two public datasets and evaluated using a set of performance evaluation measures. In these experiments, the accuracy result is compared with the accuracies of baseline ML classifiers in the recent works. Experimental results reveal that the proposed model can avert the uncertainty in selection the values of RF’s parameters, improving the accuracy of anomaly classification in NIDSs without incurring excessive time.Keywords
Cite This Article
Citations
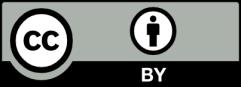
This work is licensed under a Creative Commons Attribution 4.0 International License , which permits unrestricted use, distribution, and reproduction in any medium, provided the original work is properly cited.