Open Access
ARTICLE
Recommender Systems Based on Tensor Decomposition
1 School of Information Engineering, Nanjing Audit University, Nanjing, 211815, China
2 School of Computing, National University of Singapore, 117417, Singapore
3 Jiangsu Key Laboratory of Data Science and Smart Software, Jinling Institute of Technology, Nanjing, 211169, China
* Corresponding Author: Zhoubao Sun. Email:
Computers, Materials & Continua 2021, 66(1), 621-630. https://doi.org/10.32604/cmc.2020.012593
Received 05 July 2020; Accepted 31 July 2020; Issue published 30 October 2020
Abstract
Recommender system is an effective tool to solve the problems of information overload. The traditional recommender systems, especially the collaborative filtering ones, only consider the two factors of users and items. While social networks contain abundant social information, such as tags, places and times. Researches show that the social information has a great impact on recommendation results. Tags not only describe the characteristics of items, but also reflect the interests and characteristics of users. Since the traditional recommender systems cannot parse multi-dimensional information, in this paper, a tensor decomposition model based on tag regularization is proposed which incorporates social information to benefit recommender systems. The original Singular Value Decomposition (SVD) model is optimized by mining the co-occurrence and mutual exclusion of tags, and their features are constrained by the relationship between tags. Experiments on real dataset show that the proposed algorithm achieves superior performance to existing algorithms.Keywords
Cite This Article
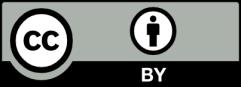
This work is licensed under a Creative Commons Attribution 4.0 International License , which permits unrestricted use, distribution, and reproduction in any medium, provided the original work is properly cited.