Open Access
ARTICLE
Smart Healthcare Using Data-Driven Prediction of Immunization Defaulters in Expanded Program on Immunization (EPI)
1 Predicitive Analytics Laboratory, Shaheed Zulfikar Ali Bhutto Institute of Science and Technology, Islamabad, 44000, Pakistan
2 Department of Software Engineering, Foundation University Islamabad, Islamabad, 44000, Pakistan
3 Department of Software, Sejong University, Seoul, 05006, Korea
4 Department of Computer Science and Engineering, Soonchunhyang University, Asan, 31538, Korea
* Corresponding Author: Yunyoung Nam. Email:
(This article belongs to the Special Issue: Artificial Intelligence and IoT based intelligent systems using high performance computing for Medical applications.)
Computers, Materials & Continua 2021, 66(1), 589-602. https://doi.org/10.32604/cmc.2020.012507
Received 02 July 2020; Accepted 26 July 2020; Issue published 30 October 2020
Abstract
Immunization is a noteworthy and proven tool for eliminating lifethreating infectious diseases, child mortality and morbidity. Expanded Program on Immunization (EPI) is a nation-wide program in Pakistan to implement immunization activities, however the coverage is quite low despite the accessibility of free vaccination. This study proposes a defaulter prediction model for accurate identification of defaulters. Our proposed framework classifies defaulters at five different stages: defaulter, partially high, partially medium, partially low, and unvaccinated to reinforce targeted interventions by accurately predicting children at high risk of defaulting from the immunization schedule. Different machine learning algorithms are applied on Pakistan Demographic and Health Survey (2017–18) dataset. Multilayer Perceptron yielded 98.5% accuracy for correctly identifying children who are likely to default from immunization series at different risk stages of being defaulter. In this paper, the proposed defaulters’ prediction framework is a step forward towards a data-driven approach and provides a set of machine learning techniques to take advantage of predictive analytics. Hence, predictive analytics can reinforce immunization programs by expediting targeted action to reduce dropouts. Specially, the accurate predictions support targeted messages sent to at-risk parents’ and caretakers’ consumer devices (e.g., smartphones) to maximize healthcare outcomes.Keywords
Cite This Article
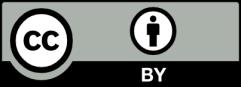
This work is licensed under a Creative Commons Attribution 4.0 International License , which permits unrestricted use, distribution, and reproduction in any medium, provided the original work is properly cited.