Open Access
ARTICLE
A Rasterized Lightning Disaster Risk Method for Imbalanced Sets Using Neural Network
1 Nanjing University of Information Science & Technology, Nanjing, 210044, China
2 Engineering Research Center of Digital Forensics, Ministry of Education, Nanjing, 201144, China
3 Waterford Institute of Technology, Waterford, X91K0EK, Ireland
* Corresponding Author: Jin Han. Email:
Computers, Materials & Continua 2021, 66(1), 563-574. https://doi.org/10.32604/cmc.2020.012502
Received 02 July 2020; Accepted 10 September 2020; Issue published 30 October 2020
Abstract
Over the past 10 years, lightning disaster has caused a large number of casualties and considerable economic loss worldwide. Lightning poses a huge threat to various industries. In an attempt to reduce the risk of lightning-caused disaster, many scholars have carried out in-depth research on lightning. However, these studies focus primarily on the lightning itself and other meteorological elements are ignored. In addition, the methods for assessing the risk of lightning disaster fail to give detailed attention to regional features (lightning disaster risk). This paper proposes a grid-based risk assessment method based on data from multiple sources. First, this paper considers the impact of lightning, the population density, the economy, and geographical environment data on the occurrence of lightning disasters; Second, this paper solves the problem of imbalanced lightning disaster data in geographic grid samples based on the K-means clustering algorithm; Third, the method calculates the feature of lightning disaster in each small field with the help of neural network structure, and the calculation results are then visually reflected in a zoning map by the Jenks natural breaks algorithm. The experimental results show that our method can solve the problem of imbalanced lightning disaster data, and offer 81% accuracy in lightning disaster risk assessment.Keywords
Cite This Article
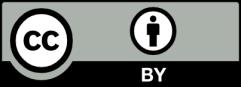
This work is licensed under a Creative Commons Attribution 4.0 International License , which permits unrestricted use, distribution, and reproduction in any medium, provided the original work is properly cited.