Open Access
ARTICLE
Enhance Intrusion Detection in Computer Networks Based on Deep Extreme Learning Machine
1 Department of Computer Science, Lahore Garrison University, Lahore, 54792, Pakistan
2 School of Computer Science, NCBA&E, Lahore, 54000, Pakistan
3 Computer Science Department, Umm Al-Qura University, Makkah City, 715, Saudi Arabia
* Corresponding Author: Muhammad Adnan Khan. Email:
Computers, Materials & Continua 2021, 66(1), 467-480. https://doi.org/10.32604/cmc.2020.013121
Received 27 July 2020; Accepted 14 August 2020; Issue published 30 October 2020
Abstract
Networks provide a significant function in everyday life, and cybersecurity therefore developed a critical field of study. The Intrusion detection system (IDS) becoming an essential information protection strategy that tracks the situation of the software and hardware operating on the network. Notwithstanding advancements of growth, current intrusion detection systems also experience dif- ficulties in enhancing detection precision, growing false alarm levels and identifying suspicious activities. In order to address above mentioned issues, several researchers concentrated on designing intrusion detection systems that rely on machine learning approaches. Machine learning models will accurately identify the underlying variations among regular information and irregular information with incredible efficiency. Artificial intelligence, particularly machine learning methods can be used to develop an intelligent intrusion detection framework. There in this article in order to achieve this objective, we propose an intrusion detection system focused on a Deep extreme learning machine (DELM) which first establishes the assessment of safety features that lead to their prominence and then constructs an adaptive intrusion detection system focusing on the important features. In the moment, we researched the viability of our suggested DELMbased intrusion detection system by conducting dataset assessments and evaluating the performance factors to validate the system reliability. The experimental results illustrate that the suggested framework outclasses traditional algorithms. In fact, the suggested framework is not only of interest to scientific research but also of functional importance.Keywords
Cite This Article
Citations
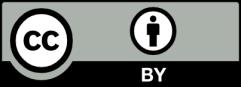
This work is licensed under a Creative Commons Attribution 4.0 International License , which permits unrestricted use, distribution, and reproduction in any medium, provided the original work is properly cited.