Open Access
ARTICLE
Deep Feature Extraction and Feature Fusion for Bi-Temporal Satellite Image Classification
1 Department of ECE, Karunya Institute of Technology and Sciences, Coimbatore, India
2 Department of Computer Science, Alexandru Ioan Cuza University of laşi, laşi, Romania
3 Department of Computer Science, University of Craiovo, Craiovo, Romania
* Corresponding Author: D. Jude Hemanth. Email:
(This article belongs to the Special Issue: Deep Learning Trends in Intelligent Systems)
Computers, Materials & Continua 2021, 66(1), 373-388. https://doi.org/10.32604/cmc.2020.012364
Received 27 June 2020; Accepted 26 July 2020; Issue published 30 October 2020
Abstract
Multispectral images contain a large amount of spatial and spectral data which are effective in identifying change areas. Deep feature extraction is important for multispectral image classification and is evolving as an interesting research area in change detection. However, many deep learning framework based approaches do not consider both spatial and textural details into account. In order to handle this issue, a Convolutional Neural Network (CNN) based multi-feature extraction and fusion is introduced which considers both spatial and textural features. This method uses CNN to extract the spatio-spectral features from individual channels and fuse them with the textural features. Then the fused image is classified into change and unchanged regions. The presence of mixed pixels in the bitemporal satellite images affect the classification accuracy due to the misclassification errors. The proposed method was compared with six state-of-theart change detection methods and analyzed. The main highlight of this method is that by taking into account the spatio-spectral and textural information in the input channels, the mixed pixel problem is solved. Experiments indicate the effectiveness of this method and demonstrate that it possesses low misclassification errors, higher overall accuracy and kappa coefficient.Keywords
Cite This Article
Citations
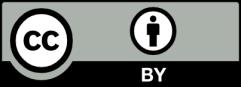
This work is licensed under a Creative Commons Attribution 4.0 International License , which permits unrestricted use, distribution, and reproduction in any medium, provided the original work is properly cited.