Open Access
ARTICLE
Prediction of COVID-19 Confirmed Cases Using Gradient Boosting Regression Method
1 College of Computer and Information Sciences, King Saud University, Riyadh, 11362, Saudi Arabia
2 Computer Science Department, Faculty of Applied Science, Taiz University, Taiz, Yemen
3 College of Applied Computer Sciences, King Saud University, Riyadh, 11362, Saudi Arabia
* Corresponding Author: Abdu Gumaei. Email:
Computers, Materials & Continua 2021, 66(1), 315-329. https://doi.org/10.32604/cmc.2020.012045
Received 11 June 2020; Accepted 22 July 2020; Issue published 30 October 2020
Abstract
The fast spread of coronavirus disease (COVID-19) caused by SARSCoV-2 has become a pandemic and a serious threat to the world. As of May 30, 2020, this disease had infected more than 6 million people globally, with hundreds of thousands of deaths. Therefore, there is an urgent need to predict confirmed cases so as to analyze the impact of COVID-19 and practice readiness in healthcare systems. This study uses gradient boosting regression (GBR) to build a trained model to predict the daily total confirmed cases of COVID-19. The GBR method can minimize the loss function of the training process and create a single strong learner from weak learners. Experiments are conducted on a dataset of daily confirmed COVID-19 cases from January 22, 2020, to May 30, 2020. The results are evaluated on a set of evaluation performance measures using 10-fold cross-validation to demonstrate the effectiveness of the GBR method. The results reveal that the GBR model achieves 0.00686 root mean square error, the lowest among several comparative models.Keywords
Cite This Article
Citations
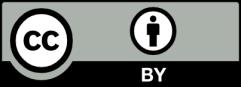
This work is licensed under a Creative Commons Attribution 4.0 International License , which permits unrestricted use, distribution, and reproduction in any medium, provided the original work is properly cited.