Open Access
ARTICLE
Straw Segmentation Algorithm Based on Modified UNet in Complex Farmland Environment
1 College of Information Technology, Jilin Agriculture University, Changchun, 130118, China
2 Department of Biosystems and Agricultural Engineering, Oklahoma State University, Stillwater, 74078, USA
3 Key Laboratory of Bionic Engineering, Ministry of Education, Jilin University, Changchun, 130025, China
4 College of Engineering and Technology, Jilin Agricultural University, Changchun, 130118, China
* Corresponding Author: Yueyong Wang. Email:
Computers, Materials & Continua 2021, 66(1), 247-262. https://doi.org/10.32604/cmc.2020.012328
Received 25 June 2020; Accepted 22 July 2020; Issue published 30 October 2020
Abstract
Intelligent straw coverage detection plays an important role in agricultural production and the ecological environment. Traditional pattern recognition has some problems, such as low precision and a long processing time, when segmenting complex farmland, which cannot meet the conditions of embedded equipment deployment. Based on these problems, we proposed a novel deep learning model with high accuracy, small model size and fast running speed named Residual Unet with Attention mechanism using depthwise convolution (RADw–UNet). This algorithm is based on the UNet symmetric codec model. All the feature extraction modules of the network adopt the residual structure, and the whole network only adopts 8 times the downsampling rate to reduce the redundant parameters. To better extract the semantic information of the spatial and channel dimensions, the depthwise convolutional residual block is designed to be used in feature maps with larger depths to reduce the number of parameters while improving the model accuracy. Meanwhile, the multi–level attention mechanism is introduced in the skip connection to effectively integrate the information of the low–level and high–level feature maps. The experimental results showed that the segmentation performance of RADw–UNet outperformed traditional methods and the UNet algorithm. The algorithm achieved an mIoU of 94.9%, the number of trainable parameters was only approximately 0.26 M, and the running time for a single picture was less than 0.03 s.Keywords
Cite This Article
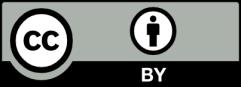
This work is licensed under a Creative Commons Attribution 4.0 International License , which permits unrestricted use, distribution, and reproduction in any medium, provided the original work is properly cited.