Open Access
ARTICLE
Detecting Lumbar Implant and Diagnosing Scoliosis from Vietnamese X-Ray Imaging Using the Pre-Trained API Models and Transfer Learning
1 Duy Tan University, Da Nang, 550000, Vietnam
2 Department of Radiology, Hue University of Medicine and Pharmacy, Hue University, Hue, 530000, Vietnam
3 Institute of Research and Development, Duy Tan University, Danang, 550000, Vietnam
4 Faculty of Information Technology, Duy Tan University, Danang, 550000, Vietnam
* Corresponding Author: Dac-Nhuong Le. Email:
Computers, Materials & Continua 2021, 66(1), 17-33. https://doi.org/10.32604/cmc.2020.013125
Received 27 July 2020; Accepted 11 September 2020; Issue published 30 October 2020
Abstract
With the rapid growth of the autonomous system, deep learning has become integral parts to enumerate applications especially in the case of healthcare systems. Human body vertebrae are the longest and complex parts of the human body. There are numerous kinds of conditions such as scoliosis, vertebra degeneration, and vertebrate disc spacing that are related to the human body vertebrae or spine or backbone. Early detection of these problems is very important otherwise patients will suffer from a disease for a lifetime. In this proposed system, we developed an autonomous system that detects lumbar implants and diagnoses scoliosis from the modified Vietnamese x-ray imaging. We applied two different approaches including pre-trained APIs and transfer learning with their pre-trained models due to the unavailability of sufficient x-ray medical imaging. The results show that transfer learning is suitable for the modified Vietnamese x-ray imaging data as compared to the pre-trained API models. Moreover, we also explored and analyzed four transfer learning models and two pre-trained API models with our datasets in terms of accuracy, sensitivity, and specificity.Keywords
Cite This Article
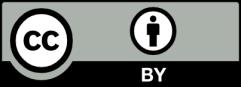
This work is licensed under a Creative Commons Attribution 4.0 International License , which permits unrestricted use, distribution, and reproduction in any medium, provided the original work is properly cited.