Open Access
ARTICLE
A Two-Stage Vehicle Type Recognition Method Combining the Most Effective Gabor Features
1 School of Automation, Nanjing University of Information Science & Technology, Nanjing, 210044, China.
2 Jiangsu Collaborative Innovation Center on Atmospheric Environment and Equipment Technology, Nanjing
University of Information Science & Technology, Nanjing, 210044, China.
3 Jiangsu Engineering Center of Network Monitoring, Nanjing University of Information Science & Technology,
Nanjing, 210044, China.
4 Rensselaer Polytechnic Institute, Troy, NY 12180, USA.
* Corresponding Author: Wei Sun. Email: .
Computers, Materials & Continua 2020, 65(3), 2489-2510. https://doi.org/10.32604/cmc.2020.012343
Received 26 June 2020; Accepted 22 July 2020; Issue published 16 September 2020
Abstract
Vehicle type recognition (VTR) is an important research topic due to its significance in intelligent transportation systems. However, recognizing vehicle type on the real-world images is challenging due to the illumination change, partial occlusion under real traffic environment. These difficulties limit the performance of current stateof-art methods, which are typically based on single-stage classification without considering feature availability. To address such difficulties, this paper proposes a twostage vehicle type recognition method combining the most effective Gabor features. The first stage leverages edge features to classify vehicles by size into big or small via a similarity k-nearest neighbor classifier (SKNNC). Further the more specific vehicle type such as bus, truck, sedan or van is recognized by the second stage classification, which leverages the most effective Gabor features extracted by a set of Gabor wavelet kernels on the partitioned key patches via a kernel sparse representation-based classifier (KSRC). A verification and correction step based on minimum residual analysis is proposed to enhance the reliability of the VTR. To improve VTR efficiency, the most effective Gabor features are selected through gray relational analysis that leverages the correlation between Gabor feature image and the original image. Experimental results demonstrate that the proposed method not only improves the accuracy of VTR but also enhances the recognition robustness to illumination change and partial occlusion.Keywords
Cite This Article
Citations
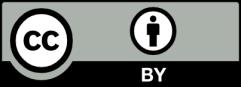
This work is licensed under a Creative Commons Attribution 4.0 International License , which permits unrestricted use, distribution, and reproduction in any medium, provided the original work is properly cited.