Open Access
ARTICLE
MOOC Learner’s Final Grade Prediction Based on an Improved Random Forests Method
1 College of Economics and Management, Nanjing University of Aeronautics and Astronautics, Nanjing,
211106, China.
2 School of Computer Science and Engineering, Nanjing University of Science and Technology, Nanjing,
210094, China.
3 Office of International Cooperation and Exchanges, Nanjing University of Finance & Economics, Nanjing,
210046, China.
4 Jiangsu Guidgine Educational Evaluation Inc., Nanjing, 210046, China.
5 Department of Electrical and Computer Engineering, University of California, Davis, CA 95616, USA.
* Corresponding Author: Peng Fu. Email: .
Computers, Materials & Continua 2020, 65(3), 2413-2423. https://doi.org/10.32604/cmc.2020.011881
Received 03 June 2020; Accepted 07 July 2020; Issue published 16 September 2020
Abstract
Massive Open Online Course (MOOC) has become a popular way of online learning used across the world by millions of people. Meanwhile, a vast amount of information has been collected from the MOOC learners and institutions. Based on the educational data, a lot of researches have been investigated for the prediction of the MOOC learner’s final grade. However, there are still two problems in this research field. The first problem is how to select the most proper features to improve the prediction accuracy, and the second problem is how to use or modify the data mining algorithms for a better analysis of the MOOC data. In order to solve these two problems, an improved random forests method is proposed in this paper. First, a hybrid indicator is defined to measure the importance of the features, and a rule is further established for the feature selection; then, a Clustering-Synthetic Minority Over-sampling Technique (SMOTE) is embedded into the traditional random forests algorithm to solve the class imbalance problem. In experiment part, we verify the performance of the proposed method by using the Canvas Network Person-Course (CNPC) dataset. Furthermore, four well-known prediction methods have been applied for comparison, where the superiority of our method has been proved.Keywords
Cite This Article
Citations
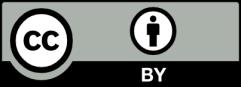