Open Access
ARTICLE
MoTransFrame: Model Transfer Framework for CNNs on Low-Resource Edge Computing Node
1 National University of Defense Technology, Changsha, 410073, China.
2 Training and Administration Department, the Central Military Commission, Beijing, 100851, China.
3 Department of Science and Technology, China Electronics Technology Group Corporation, Beijing, 100846, China.
4 National Engineering Laboratory for Public Safety Risk Perception and Control by Big Data, Beijing,
100041, China.
5 School of Design, Hunan University, Changsha, 410082, China.
6 Department of Electrical and Computer Engineering, University of Louisville, Louisville, KY 40292, USA.
* Corresponding Author: Yangyang Li. Email: .
Computers, Materials & Continua 2020, 65(3), 2321-2334. https://doi.org/10.32604/cmc.2020.010522
Received 08 March 2020; Accepted 17 July 2020; Issue published 16 September 2020
Abstract
Deep learning technology has been widely used in computer vision, speech recognition, natural language processing, and other related fields. The deep learning algorithm has high precision and high reliability. However, the lack of resources in the edge terminal equipment makes it difficult to run deep learning algorithms that require more memory and computing power. In this paper, we propose MoTransFrame, a general model processing framework for deep learning models. Instead of designing a model compression algorithm with a high compression ratio, MoTransFrame can transplant popular convolutional neural networks models to resources-starved edge devices promptly and accurately. By the integration method, Deep learning models can be converted into portable projects for Arduino, a typical edge device with limited resources. Our experiments show that MoTransFrame has good adaptability in edge devices with limited memories. It is more flexible than other model transplantation methods. It can keep a small loss of model accuracy when the number of parameters is compressed by tens of times. At the same time, the computational resources needed in the reasoning process are less than what the edge node could handle.Keywords
Cite This Article
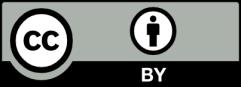