Open Access
ARTICLE
Research on Prediction Methods of Prevalence Perception under Information Exposure
1 School of Computer and Information Engineering, Hunan University of Technology and Business,
Changsha, 410205, China.
2 Key Laboratory of Hunan Province for New Retail Virtual Reality Technology, Hunan University of
Technology and Business, Changsha, 410205, China.
3 Institute of Big Data and Internet Innovation, Mobile E-Business Collaborative Innovation Center of Hunan
Province, Hunan University of Technology and Business, Changsha, 410205, China.
4 School of Computer Science and Technology, Wuhan University of Technology, Wuhan, 430073, China.
5 School of Bioinformatics, University of Minnesota, Twin Cities, USA.
* Corresponding Author: Fang Ye. Email: .
Computers, Materials & Continua 2020, 65(3), 2263-2275. https://doi.org/10.32604/cmc.2020.010082
Received 10 February 2020; Accepted 29 May 2020; Issue published 16 September 2020
Abstract
With the rapid development of information technology, the explosive growth of data information has become a common challenge and opportunity. Social network services represented by WeChat, Weibo and Twitter, drive a large amount of information due to the continuous spread, evolution and emergence of users through these platforms. The dynamic modeling, analysis, and network information prediction, has very important research and application value, and plays a very important role in the discovery of popular events, personalized information recommendation, and early warning of bad information. For these reasons, this paper proposes an adaptive prediction algorithm for network information transmission. A popularity prediction algorithm is designed to control the transmission trend based on the gray Verhulst model to analyze the law of development and capture popular trends. Experimental simulations show that the proposed perceptual prediction model in this paper has a better fitting effect than the existing models.Keywords
Cite This Article
Citations
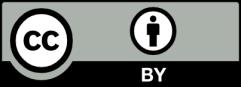
This work is licensed under a Creative Commons Attribution 4.0 International License , which permits unrestricted use, distribution, and reproduction in any medium, provided the original work is properly cited.