Open Access
ARTICLE
Road Damage Detection and Classification Using Mask R-CNN with DenseNet Backbone
1 School of Computer and Communication Engineering, Zhengzhou University of Light Industry, Zhengzhou,
450000, China.
2 SIPPR Engineering Group Co., Ltd., Zhengzhou, 450000, China.
3 College of Electronic and Electrical Engineering, Sungkyunkwan University, Suwon, 440746, Korea.
* Corresponding Author: Qiqiang Chen. Email: .
Computers, Materials & Continua 2020, 65(3), 2201-2215. https://doi.org/10.32604/cmc.2020.011191
Received 24 April 2020; Accepted 08 June 2020; Issue published 16 September 2020
Abstract
Automatic road damage detection using image processing is an important aspect of road maintenance. It is also a challenging problem due to the inhomogeneity of road damage and complicated background in the road images. In recent years, deep convolutional neural network based methods have been used to address the challenges of road damage detection and classification. In this paper, we propose a new approach to address those challenges. This approach uses densely connected convolution networks as the backbone of the Mask R-CNN to effectively extract image feature, a feature pyramid network for combining multiple scales features, a region proposal network to generate the road damage region, and a fully convolutional neural network to classify the road damage region and refine the region bounding box. This method can not only detect and classify the road damage, but also create a mask of the road damage. Experimental results show that the proposed approach can achieve better results compared with other existing methods.Keywords
Cite This Article
Citations
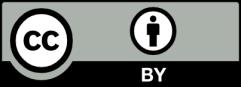
This work is licensed under a Creative Commons Attribution 4.0 International License , which permits unrestricted use, distribution, and reproduction in any medium, provided the original work is properly cited.