Open Access
ARTICLE
Research on the Freezing Phenomenon of Quantum Correlation by Machine Learning
1 School of Information and Software Engineering, University of Electronic Science and Technology of China,
Chengdu, 610054, China.
2 School of Physics, University of Electronic Science and Technology of China, Chengdu, 610054, China.
3 Department of Chemistry and Biochemistry, Utah State University, Logan, Utah, 84322, USA.
* Corresponding Author: Qinsheng Zhu. Email: .
Computers, Materials & Continua 2020, 65(3), 2143-2151. https://doi.org/10.32604/cmc.2020.010865
Received 02 April 2020; Accepted 28 June 2020; Issue published 16 September 2020
Abstract
Quantum correlation shows a fascinating nature of quantum mechanics and plays an important role in some physics topics, especially in the field of quantum information. Quantum correlations of the composite system can be quantified by resorting to geometric or entropy methods, and all these quantification methods exhibit the peculiar freezing phenomenon. The challenge is to find the characteristics of the quantum states that generate the freezing phenomenon, rather than only study the conditions which generate this phenomenon under a certain quantum system. In essence, this is a classification problem. Machine learning has become an effective method for researchers to study classification and feature generation. In this work, we prove that the machine learning can solve the problem of X form quantum states, which is a problem of physical significance. Subsequently, we apply the density-based spatial clustering of applications with noise (DBSCAN) algorithm and the decision tree to divide quantum states into two different groups. Our goal is to classify the quantum correlations of quantum states into two classes: one is the quantum correlation with freezing phenomenon for both Rènyi discord (Keywords
Cite This Article
Citations
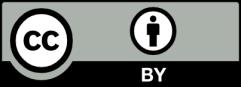