Open Access
ARTICLE
An Early Stopping-Based Artificial Neural Network Model for Atmospheric Corrosion Prediction of Carbon Steel
1 National Center for Materials Service Safety, University of Science and Technology Beijing, Beijing,
100083, China.
2 Department of Computer Engineering and Information Technology, Yangon Technological University,
Yangon, 11181, Myanmar.
* Corresponding Author: Peng Shi. Email: .
Computers, Materials & Continua 2020, 65(3), 2091-2109. https://doi.org/10.32604/cmc.2020.011608
Received 19 May 2020; Accepted 21 June 2020; Issue published 16 September 2020
Abstract
The optimization of network topologies to retain the generalization ability by deciding when to stop overtraining an artificial neural network (ANN) is an existing vital challenge in ANN prediction works. The larger the dataset the ANN is trained with, the better generalization the prediction can give. In this paper, a large dataset of atmospheric corrosion data of carbon steel compiled from several resources is used to train and test a multilayer backpropagation ANN model as well as two conventional corrosion prediction models (linear and Klinesmith models). Unlike previous related works, a grid searchbased hyperparameter tuning is performed to develop multiple hyperparameter combinations (network topologies) to train multiple ANNs with mini-batch stochastic gradient descent optimization algorithm to facilitate the training of a large dataset. After that, one selection strategy for the optimal hyperparameter combination is applied by an early stopping method to guarantee the generalization ability of the optimal network model. The correlation coefficients (R) of the ANN model can explain about 80% (more than 75%) of the variance of atmospheric corrosion of carbon steel, and the root mean square errors (RMSE) of three models show that the ANN model gives a better performance than the other two models with acceptable generalization. The influence of input parameters on the output is highlighted by using the fuzzy curve analysis method. The result reveals that TOW, Cl- and SO2 are the most important atmospheric chemical variables, which have a well-known nonlinear relationship with atmospheric corrosion.Keywords
Cite This Article
Citations
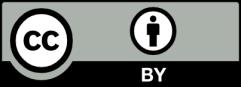
This work is licensed under a Creative Commons Attribution 4.0 International License , which permits unrestricted use, distribution, and reproduction in any medium, provided the original work is properly cited.