Open Access
ARTICLE
Millimeter-Wave Concurrent Beamforming: A Multi-Player Multi-Armed Bandit Approach
1 Electrical Engineering Department, College of Engineering, Prince Sattam Bin Abdulaziz University, Wadi
Addwasir, 11991, Saudi Arabia.
2 Electrical Engineering Department, Faculty of Engineering, Aswan University, Aswan, 81542, Egypt.
3 Computational Learning Theory Team, RIKEN-Advanced Intelligent Project, Fukuoka, 819-0395, Japan.
4 Engineering Department, Nuclear Research Center, Egyptian Atomic Energy Authority, Cairo, 13759, Egypt.
5 Faculty of Arts and Science, Kyushu University, Fukuok, 819-0395, Japan.
6 Electronics and Electrical Communication Engineering, Faculty of Electronic Engineering, Menoufia University, Menouf, 32952, Egypt.
* Corresponding Author: Ehab Mahmoud Mohamed. Email: .
Computers, Materials & Continua 2020, 65(3), 1987-2007. https://doi.org/10.32604/cmc.2020.011816
Received 30 May 2020; Accepted 10 July 2020; Issue published 16 September 2020
Abstract
The communication in the Millimeter-wave (mmWave) band, i.e., 30~300 GHz, is characterized by short-range transmissions and the use of antenna beamforming (BF). Thus, multiple mmWave access points (APs) should be installed to fully cover a target environment with gigabits per second (Gbps) connectivity. However, inter-beam interference prevents maximizing the sum rates of the established concurrent links. In this paper, a reinforcement learning (RL) approach is proposed for enabling mmWave concurrent transmissions by finding out beam directions that maximize the long-term average sum rates of the concurrent links. Specifically, the problem is formulated as a multiplayer multiarmed bandit (MAB), where mmWave APs act as the players aiming to maximize their achievable rewards, i.e., data rates, and the arms to play are the available beam directions. In this setup, a selfish concurrent multiplayer MAB strategy is advocated. Four different MAB algorithms, namely, ϵ-greedy, upper confidence bound (UCB), Thompson sampling (TS), and exponential weight algorithm for exploration and exploitation (EXP3) are examined by employing them in each AP to selfishly enhance its beam selection based only on its previous observations. After a few rounds of interactions, mmWave APs learn how to select concurrent beams that enhance the overall system performance. The proposed MAB based mmWave concurrent BF shows comparable performance to the optimal solution.Keywords
Cite This Article
Citations
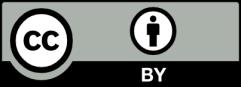
This work is licensed under a Creative Commons Attribution 4.0 International License , which permits unrestricted use, distribution, and reproduction in any medium, provided the original work is properly cited.