Open Access
ARTICLE
Study on Multi-Label Classification of Medical Dispute Documents
1 School of Computer Science and Engineering, Southeast University, Nanjing, 21189, China.
2 Key Laboratory of Computer Network and Information Integration of Ministry of Education, Southeast
University, Nanjing, 211189, China.
3 Research Center for Judicial Big Data, Supreme Count of China, Nanjing, 211189, China.
4 University of Lincoln, Lincoln, LN6 7TS, UK.
* Corresponding Author: Baili Zhang. Email: .
Computers, Materials & Continua 2020, 65(3), 1975-1986. https://doi.org/10.32604/cmc.2020.010914
Received 06 April 2020; Accepted 17 July 2020; Issue published 16 September 2020
Abstract
The Internet of Medical Things (IoMT) will come to be of great importance in the mediation of medical disputes, as it is emerging as the core of intelligent medical treatment. First, IoMT can track the entire medical treatment process in order to provide detailed trace data in medical dispute resolution. Second, IoMT can infiltrate the ongoing treatment and provide timely intelligent decision support to medical staff. This information includes recommendation of similar historical cases, guidance for medical treatment, alerting of hired dispute profiteers etc. The multi-label classification of medical dispute documents (MDDs) plays an important role as a front-end process for intelligent decision support, especially in the recommendation of similar historical cases. However, MDDs usually appear as long texts containing a large amount of redundant information, and there is a serious distribution imbalance in the dataset, which directly leads to weaker classification performance. Accordingly, in this paper, a multi-label classification method based on key sentence extraction is proposed for MDDs. The method is divided into two parts. First, the attention-based hierarchical bi-directional long short-term memory (BiLSTM) model is used to extract key sentences from documents; second, random comprehensive sampling Bagging (RCS-Bagging), which is an ensemble multi-label classification model, is employed to classify MDDs based on key sentence sets. The use of this approach greatly improves the classification performance. Experiments show that the performance of the two models proposed in this paper is remarkably better than that of the baseline methods.Keywords
Cite This Article
Citations
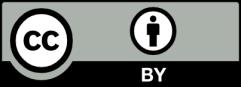
This work is licensed under a Creative Commons Attribution 4.0 International License , which permits unrestricted use, distribution, and reproduction in any medium, provided the original work is properly cited.