Open Access
ARTICLE
Analysis of Feature Importance and Interpretation for Malware Classification
1 Department of Computer Engineering, Gachon University, Sungnam-si, 13120, Korea.
2 Department of Software, Gachon University, Sungnam-si, 13120, Korea.
* Corresponding Author: Myung-Mook Han. Email: .
Computers, Materials & Continua 2020, 65(3), 1891-1904. https://doi.org/10.32604/cmc.2020.010933
Received 08 April 2020; Accepted 28 July 2020; Issue published 16 September 2020
Abstract
This study was conducted to enable prompt classification of malware, which was becoming increasingly sophisticated. To do this, we analyzed the important features of malware and the relative importance of selected features according to a learning model to assess how those important features were identified. Initially, the analysis features were extracted using Cuckoo Sandbox, an open-source malware analysis tool, then the features were divided into five categories using the extracted information. The 804 extracted features were reduced by 70% after selecting only the most suitable ones for malware classification using a learning model-based feature selection method called the recursive feature elimination. Next, these important features were analyzed. The level of contribution from each one was assessed by the Random Forest classifier method. The results showed that System call features were mostly allocated. At the end, it was possible to accurately identify the malware type using only 36 to 76 features for each of the four types of malware with the most analysis samples available. These were the Trojan, Adware, Downloader, and Backdoor malware.Keywords
Cite This Article
Citations
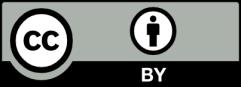