Open Access
ARTICLE
Research on Data Extraction and Analysis of Software Defect in IoT Communication Software
1 School of Computer and Software, Dalian Neusoft University of Information, Dalian, 116023, China.
2 School of Information Engineering, Qingdao Bin Hai University, Qingdao, 266555, China.
3 School of Internet of Things and Software Technology, Wuxi Vocational College of Science and
Technology, Wuxi, 214028, China.
4 Public Teaching Department, Neuedu Software Talent Training School, Qingdao, 266000, China.
5 Public Teaching Department, Qingdao Technical College, Qingdao, 266555, China.
6 School of Information Engineering, Sanming University, Sanming, 365004, China.
7 School of Mathematics and Statistics, University College Dublin, Dublin, Ireland.
* Corresponding Author: Guangsheng Cao. Email: .
Computers, Materials & Continua 2020, 65(2), 1837-1854. https://doi.org/10.32604/cmc.2020.010420
Received 04 March 2020; Accepted 02 May 2020; Issue published 20 August 2020
Abstract
Software defect feature selection has problems of feature space dimensionality reduction and large search space. This research proposes a defect prediction feature selection framework based on improved shuffled frog leaping algorithm (ISFLA).Using the two-level structure of the framework and the improved hybrid leapfrog algorithm's own advantages, the feature values are sorted, and some features with high correlation are selected to avoid other heuristic algorithms in the defect prediction that are easy to produce local The case where the convergence rate of the optimal or parameter optimization process is relatively slow. The framework improves generalization of predictions of unknown data samples and enhances the ability to search for features related to learning tasks. At the same time, this framework further reduces the dimension of the feature space. After the contrast simulation experiment with other common defect prediction methods, we used the actual test data set to verify the framework for multiple iterations on Internet of Things (IoT) system platform. The experimental results show that the software defect prediction feature selection framework based on ISFLA is very effective in defect prediction of IoT communication software. This framework can save the testing time of IoT communication software, effectively improve the performance of software defect prediction, and ensure the software quality.Keywords
Cite This Article
Citations
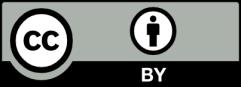
This work is licensed under a Creative Commons Attribution 4.0 International License , which permits unrestricted use, distribution, and reproduction in any medium, provided the original work is properly cited.