Open Access
ARTICLE
Recommendation Algorithm Based on Probabilistic Matrix Factorization with Adaboost
1 College of Computer Science and Technology, Jilin University, Changchun, 130012, China.
2 Symbol Computation and Knowledge Engineer of Ministry of Education, Jilin University, Changchun, 130012, China.
3 Department of Engineering Mechanics, State Marine Technical University of St. Petersburg, St. Petersburg, 190008, Russia.
* Corresponding Author: Yu Jiang. Email: .
Computers, Materials & Continua 2020, 65(2), 1591-1603. https://doi.org/10.32604/cmc.2020.09981
Received 02 February 2020; Accepted 28 June 2020; Issue published 20 August 2020
Abstract
A current problem in diet recommendation systems is the matching of food preferences with nutritional requirements, taking into account individual characteristics, such as body weight with individual health conditions, such as diabetes. Current dietary recommendations employ association rules, content-based collaborative filtering, and constraint-based methods, which have several limitations. These limitations are due to the existence of a special user group and an imbalance of non-simple attributes. Making use of traditional dietary recommendation algorithm researches, we combine the Adaboost classifier with probabilistic matrix factorization. We present a personalized diet recommendation algorithm by taking advantage of probabilistic matrix factorization via Adaboost. A probabilistic matrix factorization method extracts the implicit factors between individual food preferences and nutritional characteristics. From this, we can make use of those features with strong influence while discarding those with little influence. After incorporating these changes into our approach, we evaluated our algorithm’s performance. Our results show that our method performed better than others at matching preferred foods with dietary requirements, benefiting user health as a result. The algorithm fully considers the constraint relationship between users’ attributes and nutritional characteristics of foods. Considering many complex factors in our algorithm, the recommended food result set meets both health standards and users’ dietary preferences. A comparison of our algorithm with others demonstrated that our method offers high accuracy and interpretability.Keywords
Cite This Article
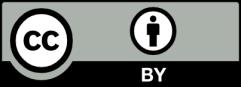
This work is licensed under a Creative Commons Attribution 4.0 International License , which permits unrestricted use, distribution, and reproduction in any medium, provided the original work is properly cited.