Open Access
ARTICLE
Adversarial Attacks on License Plate Recognition Systems
1 Cyberspace Institute of Advanced Technology, Guangzhou University, Guangzhou, 510006, China.
2 Da Hengqin Science and Technology Development Company, Ltd., Zhuhai, 519000, China.
3 Department of Computer Science, Rice University, Houston, TX 77025, USA.
* Corresponding Author: Le Wang. Email: .
Computers, Materials & Continua 2020, 65(2), 1437-1452. https://doi.org/10.32604/cmc.2020.011834
Received 31 May 2020; Accepted 16 June 2020; Issue published 20 August 2020
Abstract
The license plate recognition system (LPRS) has been widely adopted in daily life due to its efficiency and high accuracy. Deep neural networks are commonly used in the LPRS to improve the recognition accuracy. However, researchers have found that deep neural networks have their own security problems that may lead to unexpected results. Specifically, they can be easily attacked by the adversarial examples that are generated by adding small perturbations to the original images, resulting in incorrect license plate recognition. There are some classic methods to generate adversarial examples, but they cannot be adopted on LPRS directly. In this paper, we modify some classic methods to generate adversarial examples that could mislead the LPRS. We conduct extensive evaluations on the HyperLPR system and the results show that the system could be easily attacked by such adversarial examples. In addition, we show that the generated images could also attack the black-box systems; we show some examples that the Baidu LPR system also makes incorrect recognitions. We hope this paper could help improve the LPRS by realizing the existence of such adversarial attacks.Keywords
Cite This Article
Citations
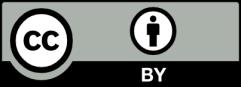
This work is licensed under a Creative Commons Attribution 4.0 International License , which permits unrestricted use, distribution, and reproduction in any medium, provided the original work is properly cited.