Open Access
ARTICLE
Robust Core Tensor Dictionary Learning with Modified Gaussian Mixture Model for Multispectral Image Restoration
1 School of Computer Science and Technology, Shandong University of Finance and Economics, Jinan, 250014, China.
2 School of Computer Science and Engineering, University of Jinan, Jinan, 250024, China.
3 School of Computer Science and Engineering, Nanyang Technological University, 639798, Singapore.
4 School of Computer Science and Engineering, Nanjing University of Science and Technology, Nanjing, 210094, China.
* Corresponding Author: Peng Fu. Email: .
Computers, Materials & Continua 2020, 65(1), 913-928. https://doi.org/10.32604/cmc.2020.09975
Received 02 February 2020; Accepted 30 April 2020; Issue published 23 July 2020
Abstract
The multispectral remote sensing image (MS-RSI) is degraded existing multispectral camera due to various hardware limitations. In this paper, we propose a novel core tensor dictionary learning approach with the robust modified Gaussian mixture model for MS-RSI restoration. First, the multispectral patch is modeled by three-order tensor and high-order singular value decomposition is applied to the tensor. Then the task of MS-RSI restoration is formulated as a minimum sparse core tensor estimation problem. To improve the accuracy of core tensor coding, the core tensor estimation based on the robust modified Gaussian mixture model is introduced into the proposed model by exploiting the sparse distribution prior in image. When applied to MS-RSI restoration, our experimental results have shown that the proposed algorithm can better reconstruct the sharpness of the image textures and can outperform several existing state-of-the-art multispectral image restoration methods in both subjective image quality and visual perception.Keywords
Cite This Article
Citations
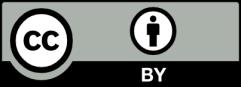
This work is licensed under a Creative Commons Attribution 4.0 International License , which permits unrestricted use, distribution, and reproduction in any medium, provided the original work is properly cited.