Open Access
ARTICLE
Automated Chinese Essay Scoring Based on Deep Learning
1 National Engineering Research Center for E-Learning, Central China Normal University, Wuhan, 430079, China.
2 School of Computer, Central China Normal University, Wuhan, 430079, China.
3 Information Retrieval and Knowledge Management Research Laboratory, Central China Normal University, Wuhan, 430079, China.
4 School of Education, South-Central University for Nationalities, Wuhan, 430074, China.
5 College of Computer Science, South-Central University for Nationalities, Wuhan, 430074, China.
6 Information School, University of Washington, Seattle, WA98105, USA.
* Corresponding Author: Tingting He. Email: .
Computers, Materials & Continua 2020, 65(1), 817-833. https://doi.org/10.32604/cmc.2020.010471
Received 06 March 2020; Accepted 02 June 2020; Issue published 23 July 2020
Abstract
Writing is an important part of language learning and is considered the best approach to demonstrate the comprehensive language skills of students. Manually grading student essays is a time-consuming task; however, it is necessary. An automated essay scoring system can not only greatly improve the efficiency of essay scoring, but also provide more objective score. Therefore, many researchers have been exploring automated essay scoring techniques and tools. However, the technique of scoring Chinese essays is still limited, and its accuracy needs to be enhanced further. To improve the accuracy of the scoring model for a Chinese essay, we propose an automated scoring approach based on a deep learning model and validate its effect by conducting two comparison experiments. The experimental results indicate that the accuracy of the proposed model is significantly higher than that of multiple linear regression (MLR), which was commonly used in the past. The three accuracy rates of the proposed model are comparable to those of the novice teacher. The root mean square error (RMSE) of the proposed model is slightly lower than that of the novice teacher, and the correlation coefficient of the proposed model is also significantly higher than that of the novice teacher. Besides, when the predicted scores are not very low or very high, the two predicted models are as good as a novice teacher. However, when the predicted score is very high or very low, the results should be treated with caution.Keywords
Cite This Article
Citations
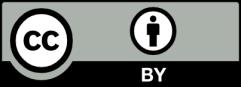