Open Access
ARTICLE
Jointly Part-of-Speech Tagging and Semantic Role Labeling Using Auxiliary Deep Neural Network Model
1 School of Computer Science and Engineering, Southeast University, Nanjing, 210000, China.
2 Henan Key Laboratory of Big Data Analysis and Processing, Henan University, Kaifeng, 475000, China.
3 Swinburne Data Science Research Institute, Swinburne University of Technology, Victoria, 3122, Australia.
* Corresponding Author: Wenke Ding. Email: .
Computers, Materials & Continua 2020, 65(1), 529-541. https://doi.org/10.32604/cmc.2020.011139
Received 22 April 2020; Accepted 20 May 2020; Issue published 23 July 2020
Abstract
Previous studies have shown that there is potential semantic dependency between part-of-speech and semantic roles. At the same time, the predicate-argument structure in a sentence is important information for semantic role labeling task. In this work, we introduce the auxiliary deep neural network model, which models semantic dependency between part-of-speech and semantic roles and incorporates the information of predicate-argument into semantic role labeling. Based on the framework of joint learning, part-of-speech tagging is used as an auxiliary task to improve the result of the semantic role labeling. In addition, we introduce the argument recognition layer in the training process of the main task-semantic role labeling, so the argument-related structural information selected by the predicate through the attention mechanism is used to assist the main task. Because the model makes full use of the semantic dependency between part-of-speech and semantic roles and the structural information of predicateargument, our model achieved the F1 value of 89.0% on the WSJ test set of CoNLL2005, which is superior to existing state-of-the-art model about 0.8%.Keywords
Cite This Article
Citations
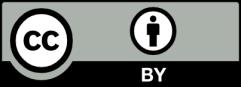
This work is licensed under a Creative Commons Attribution 4.0 International License , which permits unrestricted use, distribution, and reproduction in any medium, provided the original work is properly cited.