Open Access
ARTICLE
Image Super-Resolution Based on Generative Adversarial Networks: A Brief Review
1 School of Physics and Mechanical and Electronic Engineering, Hechi University, Yizhou, 546300, China.
2 School of Electrical and Information Engineering, Guangxi University of Science and Technology, Liuzhou,
545006, China.
3 School of Computer Science and Engineering, Hunan University of Science and Technology, Xiangtan,
411100, China.
4 School of Information Technology, Deakin University, Geelong, VIC 3220, Australia.
* Corresponding Author: Jiansheng Peng. Email: .
Computers, Materials & Continua 2020, 64(3), 1977-1997. https://doi.org/10.32604/cmc.2020.09882
Received 24 January 2020; Accepted 05 April 2020; Issue published 30 June 2020
Abstract
Single image super resolution (SISR) is an important research content in the field of computer vision and image processing. With the rapid development of deep neural networks, different image super-resolution models have emerged. Compared to some traditional SISR methods, deep learning-based methods can complete the superresolution tasks through a single image. In addition, compared with the SISR methods using traditional convolutional neural networks, SISR based on generative adversarial networks (GAN) has achieved the most advanced visual performance. In this review, we first explore the challenges faced by SISR and introduce some common datasets and evaluation metrics. Then, we review the improved network structures and loss functions of GAN-based perceptual SISR. Subsequently, the advantages and disadvantages of different networks are analyzed by multiple comparative experiments. Finally, we summarize the paper and look forward to the future development trends of GAN-based perceptual SISR.Keywords
Cite This Article
Citations
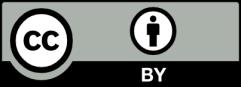