Open Access
ARTICLE
ECG Classification Using Deep CNN Improved by Wavelet Transform
1 School of Computer Science and Technology, Qilu University of Technology (Shandong Academy of
Sciences), Jinan, 250353, China.
2 Centre of Artificial Intelligence, University of Technology Sydney, Sydney, NSW 2006, Australia.
* Corresponding Author: Jinyong Cheng. Email: .
Computers, Materials & Continua 2020, 64(3), 1615-1628. https://doi.org/10.32604/cmc.2020.09938
Received 30 January 2020; Accepted 18 March 2020; Issue published 30 June 2020
Abstract
Atrial fibrillation is the most common persistent form of arrhythmia. A method based on wavelet transform combined with deep convolutional neural network is applied for automatic classification of electrocardiograms. Since the ECG signal is easily inferred, the ECG signal is decomposed into 9 kinds of subsignals with different frequency scales by wavelet function, and then wavelet reconstruction is carried out after segmented filtering to eliminate the influence of noise. A 24-layer convolution neural network is used to extract the hierarchical features by convolution kernels of different sizes, and finally the softmax classifier is used to classify them. This paper applies this method of the ECG data set provided by the 2017 PhysioNet/CINC challenge. After cross validation, this method can obtain 87.1% accuracy and the F1 score is 86.46%. Compared with the existing classification method, our proposed algorithm has higher accuracy and generalization ability for ECG signal data classification.Keywords
Cite This Article
Citations
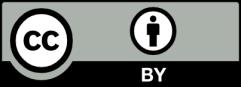
This work is licensed under a Creative Commons Attribution 4.0 International License , which permits unrestricted use, distribution, and reproduction in any medium, provided the original work is properly cited.