Open Access
ARTICLE
Intelligent Forecasting Model of COVID-19 Novel Coronavirus Outbreak Empowered with Deep Extreme Learning Machine
1 Department of Computer Science, Lahore Garrison University, Lahore, 54000, Pakistan.
2 Department of Computer Science, National College of Business Administration & Economics, Lahore,
54000, Pakistan.
3 Department of Computer Science, Umm Al-Qura University, Makkah, 23500, Saudi Arabia.
* Corresponding Author: Muhammad Adnan Khan. Email: .
(This article belongs to the Special Issue: Artificial Intelligence and Information Technologies for COVID-19)
Computers, Materials & Continua 2020, 64(3), 1329-1342. https://doi.org/10.32604/cmc.2020.011155
Received 22 April 2020; Accepted 30 April 2020; Issue published 30 June 2020
Abstract
An epidemic is a quick and widespread disease that threatens many lives and damages the economy. The epidemic lifetime should be accurate so that timely and remedial steps are determined. These include the closing of borders schools, suspension of community and commuting services. The forecast of an outbreak effectively is a very necessary but difficult task. A predictive model that provides the best possible forecast is a great challenge for machine learning with only a few samples of training available. This work proposes and examines a prediction model based on a deep extreme learning machine (DELM). This methodology is used to carry out an experiment based on the recent Wuhan coronavirus outbreak. An optimized prediction model that has been developed, namely DELM, is demonstrated to be able to make a prediction that is fairly best. The results show that the new methodology is useful in developing an appropriate forecast when the samples are far from abundant during the critical period of the disease. During the investigation, it is shown that the proposed approach has the highest accuracy rate of 97.59% with 70% of training, 30% of test and validation. Simulation results validate the prediction effectiveness of the proposed scheme.Keywords
Cite This Article
Citations
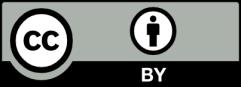